After becoming one of the first shale producers to adopt advanced data analytics, Devon Energy is now one of its most ardent champions. The results speak to why that is.
Since 2012, when Devon began investing in big data technology, its 90-day production rates from horizontal wells have increased by 250%—an improvement that according to IHS Markit data has lifted the Oklahoma City-based company’s early-time production from new wells to the highest level in the US onshore market. Additionally, Devon’s costs to drill, complete, and operate its well have fallen by as much as 40%, the company said.
It is fair to attribute some of these significant gains to the quality of the company’s positions inside North America’s most prolific shale plays, and also the pressure that oilfield contractors are under to make price concessions in a down market.
However, that does not complete the story.
From exploration and drilling to completions and production, Devon has put to work a small arsenal of sophisticated algorithms designed to find the otherwise hidden insights and engineering tweaks that are driving more value out of the shale fields it operates.
This realm of computer science has also made routine tasks that once took engineers hours to complete possible to do in a few minutes, or less. And that some of these programs can literally think for themselves, although in a limited mathematical sense, is not hyperbole.
Today’s offerings in artificial intelligence and machine learning technology can be trained to look for defined outcomes, or they can be set free to decide on their own what to look for and will rewrite their underlying algorithms in order to do so.
Humans Still in the Loop
As intriguing as this emerging world of intelligent software is, when discussing how it has changed her company, Kathy Ball, manager of advanced analytics and data science at Devon, begins by stressing the importance of the human element.
“It is about culture and collaboration,” she insisted, adding that those two ingredients constitute the “secret sauce that makes this all work.”
In other words, implementing a sophisticated data-driven approach to field development—one that relies on real-time streaming from remote field sensors, cloud-based analytics services to crunch the data in seconds, and effective procedures on how to take action on that information—depends largely on solid teamwork and sound business decisions.
Part of this requires getting analytics experts, information technology staff, and engineering leaders from different departments to climb out of their respective silos so they can share data and develop new solutions together.
On the economic justification, one simple way to assess the value of advanced analytics was put to Ball by a completions engineer, who she quoted as saying, “If it doesn’t help us extract oil and gas from the ground, then so what?”
Ball has come to call this the “so what” test. She applies it to herself, the engineers who come to her team wanting a shiny new algorithm, and to the software vendors touting their product as the latest and greatest breakthrough in artificial intelligence.
This approach filters out the ideas not worth pursuing, while allowing engineers and data experts to focus resources on ones with the most potential to generate revenue. So far, more than a dozen analytics programs have made the grade and are now integrated into the company’s day-to-day operations.
They address several key aspects of shale development: pilot testing, well spacing, expected drilling time, geosteering, geohazard prediction, bottomhole assembly selection, real-time hydraulic fracturing risk analysis, and artificial lift optimization.
Finding the Bit
Geosteering is one area where Devon believes analytics is clearly making a difference. This drilling technology has become a favorite among shale producers because it gives them a degree of precision when tapping into relatively thin pay zones.
The downside is that geosteering tools sit some distance behind the bit and surveys are only taken every 90 ft, leaving more than 100 ft of unknown wellbore trajectory each time drilling moves forward. To overcome this data gap, Devon developed a real-time drill bit tracker that leans on artificial intelligence and machine learning to work the math and figure out a well’s true path.
Engineers at the company’s drilling center keep a watchful eye on this drilling data as it is streamed in and visualized, enabling them to call for adjustments if the bit is veering off the planned course.
“That is just priceless,” Ball said of the bit tracker. “It keeps us in a producing zone, and helps us assess the landing curve,” the critical juncture where the vertical well section gives way to the horizontal section. On its website, the company credits this program with reducing the number of unplanned sidetracks it has had to drill to get wells back on their intended trajectory.
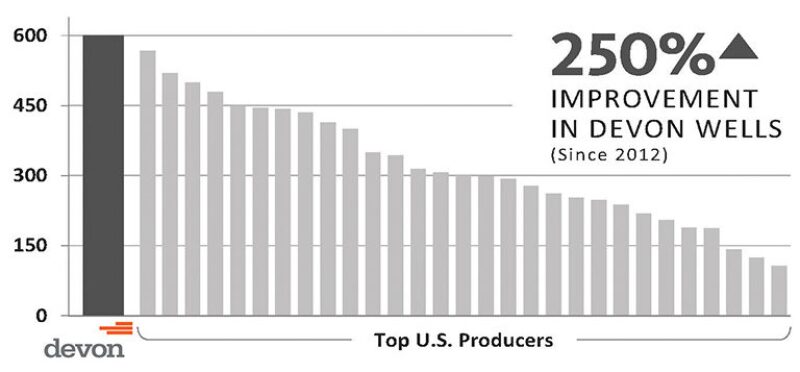
Similar analytic programs are used to model which bottomhole assembly kits will perform best in a particular well and will chart their actual performance as they rotate down the hole. Another one predicts which sections of a well are most likely to give drillers trouble (i.e., nonproductive time) and which parameters are contributing most to the rate of penetration (e.g., weight-on-bit). Taken as a whole, these drilling-focused analytics programs are preventing costly errors and helping rig crews deliver better wells in less time.
Screening Out Screenouts
Devon is also using real-time analysis to avoid screenouts—a common problem in hydraulic fracturing that happens when sand or proppant stops flowing into open fractures and begins to plug up the wellbore instead. Screenouts can be expensive to remediate and have thus encouraged the development of algorithms to detect their telltale signs.
The analysis behind Devon’s old warning system was capable of assigning risk levels between 1–4 to indicate the probability that a screenout was near. That system has since evolved into a much more deterministic model that factors in the type of fracturing fluid being used, formation characteristics, and field history.
Characterizing the role of artificial intelligence in making models like this more accurate, Ball said, “It has gone from an engineer saying, ‘Here is the pattern that you need to look for,’ to ‘All right, we have built that pattern, now let the machine make it even smarter.’”
In this case, the smarter model does away with the risk levels and instead sends engineers a 15-second warning only when a screenout is imminent. The next step is to develop a 30-second warning: plenty of time to tone down the pumping while also ensuring that maximum pressures inside the wellbore are maintained for as long as possible. Ideally, that would lead to a better stimulation treatment.
Production and Reservoir Management
One example of how analytics is giving valuable time back to engineers is an interactive map that Devon uses to calculate how much oil or gas is left in any given shale field in the US. Ball explained that an engineer tasked with doing this for 50 wells might have needed a week to do so.
“We are now able to do that through a big data solution and come up with a calculation for the Lower 48 in probably 10 minutes,” she said.
The mapping program includes data from more than 100,000 horizontal wells drilled by Devon and its competitors in the past decade. Tools such as this are allowing producers to peer over the lease line and compare their completion designs to offset operators.
For Devon, the mapping program has identified refracturing candidates and is accelerating pilot programs that seek to prove new techniques. This form of predictive analytics is also used to assess the company’s entire fleet of artificial lift units. The program determines the mean time to failure and flags lift units if the potentiality for a rod or pump failure is detected.
As a result, Devon’s field maintenance strategy has transformed from one based on a cyclical schedule to one that is largely prescriptive. Instead of field technicians inspecting a set list of wells in sequential order, the analytics program now sends them two reports a day that target only those units truly in need of attention.
“It is really important to go beyond just preventative,” Ball explained. “That will tell you that I need to replace something because it might fail—the analytics is going to tell you when it is going to fail.” She added that this program has turned out so well that it now represents one of the company’s largest areas of cost savings.
In terms of uplift, the company has noted that this combination of analytics and automation has netted single-digit percentage increases in production across its portfolio.