The oil and gas industry is at a critical juncture in addressing the pressing need to balance operational efficiency with environmental stewardship. With the world’s growing emphasis on climate change and sustainability, the industry is tasked with the dual objectives of refining operational efficiency and simultaneously diminishing its carbon footprint. This balancing act has become a strategic imperative crucial for the long-term viability of the industry. In light of the recent COP28 agreement, which emphasizes a “swift, just, and equitable energy transition,” the role of low-emission enhanced oil recovery (EOR) and operational optimization becomes increasingly significant. This period of transition, which may span several decades, demands a pragmatic approach where the existing hydrocarbon resources are used as efficiently and responsibly as possible.
In the quest to optimize operations and reduce carbon footprint, three innovative technical papers present a cohesive story of advancement through modeling and machine learning. These papers underscore the industry’s shift toward more efficient practices, each contributing a crucial piece to the larger puzzle of EOR.
The first paper introduces an advanced modeling approach for fractured reservoirs, a significant development in tight rock simulations that simplifies fracture heterogeneity characterization and enhances computational efficiency, crucial for optimizing asset development decisions in shale and tight oil resources.
Building on the theme of efficiency, the second paper pivots to the realm of surfactants, exploring their role in wettability alteration within unconventional liquid-rich reservoirs. This research provides a systematic approach for screening thermally stable surfactants under reservoir conditions, crucial for improving hydrocarbon recovery without unnecessary field trials.
The final piece of this narrative is a study using machine learning to predict gas-injection parameters in EOR processes. This approach addresses the lack of extensive gas-injection laboratory data, demonstrating the power of data-driven predictions in enhancing operational efficiency and decision-making. These papers weave a compelling narrative of technological innovation, highlighting the role of advanced modeling and machine learning.
As the oil and gas industry progresses in its vital mission to supply the world’s energy needs, the pivotal role of technology innovation becomes increasingly evident. Advanced technologies focused on operational optimization and reducing carbon emissions are essential in reinforcing the industry’s adaptability and resilience in the context of this rapidly evolving energy landscape.
This Month’s Technical Papers
Model Shows Computational Gains, Preserves Accuracy in Tight Rock EOR
Work Flow Screens Surfactants for EOR Through Wettability Alteration
Machine-Learning-Based Solution Predicts Fluid Properties for Gas-Injection Data
Recommended Additional Reading
SPE 215083 An Analytical Tool to Predict Fracture Extension and Elastic Desaturation for Polymer Field Projects by M.B. Abdullah, The University of Texas at Austin, et al.
SPE 216822 First Pilot Design of Low-Tension-Gas (LTG) Flooding in Carbonate Field in North Oman by Mohammed Al-Abri, Petroleum Development Oman, et al.
SPE 213037 Field-Scale Multistage and Multiobjective Optimization of Rate and Concentration for Polymer Flooding by Ruxin Zhang, Texas A&M University, et al.
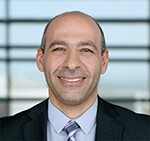
Hussein Hoteit, SPE, is a professor of earth science and engineering and chair of the Energy Resources and Petroleum Engineering program at King Abdullah University of Science and Technology (KAUST) in Saudi Arabia. Before joining KAUST in 2016, he worked for approximately 15 years for the oil and gas industry, including at Chevron and ConocoPhillips, where he conducted projects related to chemical EOR, CO2-EOR, steamflooding, and other aspects of EOR. Hoteit’s current research includes chemical EOR, waterflooding optimization, geological CO2 sequestration, CO2 mineralization in basalt, data-driven modeling, and reservoir simulation development. He has published more than 100 technical papers and has earned several SPE awards. Hoteit was an SPE Distinguished Lecturer in 2009 and earned the A Peer Apart award in 2017. He served as an associate editor for SPE Journal for more than 10 years. Hoteit holds a BS degree in mathematics from the University of Lebanon and MS and PhD degrees in computer science and applied mathematics from the University of Rennes, France.