We know that predictive models developed by artificial-intelligence (AI) and machine-learning (ML) algorithms are based on data. And, because we know how this data is used to build AI-based models, the main target of AI ethics is addressing how AI models become biased based on the quality and the quantity of the data that is used.
This second part of this two-part series discusses how AI ethics can determine and clarify how human biases of traditional engineers—assumptions, interpretations, simplifications, and preconceived notions—can be revealed in the engineering applications of AI and ML. Part 1 discussed the nonengineering applications of AI and ML and how human biases such as racism and sexism can be included in AI models through the inclusion of biased data during the training of the algorithms.
Application of AI Ethics in Engineering
Bias (including major assumptions, interpretations, and simplifications) from traditional engineers can be included in the engineering application of AI. This is usually done through the generation of data from mathematical equations, combining it with field measurements (actual physics-based data), and then using this combined set of data to model the physics using AI and ML algorithms. This approach often is called a “hybrid” model.
The ethics of AI is important to engineers and scientists who have adopted this technology to solve engineering-related problems. While AI ethics has become an important topic in the nonengineering application of AI and ML, it is just as important in engineering. The next section will present a specific example of AI ethics in the engineering application of AI and ML, showing how guesswork, assumptions, interpretations, and simplifications can lead traditional engineers using AI and ML algorithms to generate unrealistic and highly biased predictive models. This usually happens when they have tried to use facts and field measurements but were not successful.
The reasons for the inclusion of such biases in the engineering application of AI appear to be related to a lack of scientific understanding of how AI must be used to model physical phenomena. Currently, some individuals and companies who claim to be using an engineering application of this technology are including a large amount of human biases so that they can solve problems using AI after they failed to build an AI-based model that does not include human biases. Human biases in engineering have much to do with how mathematical equations are built to solve physics-based problems.
The major contribution of AI and ML to solving engineering problems is the modeling of the physical phenomena based on actual measured data, which would be the main driver for the avoidance of biases, assumptions, interpretations, and preconceived notions about physics. Because traditional techniques for modeling physical phenomena rely on mathematical equations, these techniques usually include assumptions and sometimes biases. This is especially true when the physical phenomena being modeled cannot be observed directly. Petroleum engineering is a good example of such a situation because the target hydrocarbon is deep underground. The same is true about any other engineering discipline when the mathematical equations that include assumptions, interpretations, and simplification are used to model the physical phenomena.
Reservoir engineering, reservoir modeling, and reservoir management contribute greatly to the income of the operating and service companies in the oil and gas industry. This is why reservoir modeling is so important in the petroleum industry. Modeling fluid flow in hydrocarbon reservoirs includes many assumptions, interpretations, and simplifications because the reservoirs are hundreds or thousands of feet below the surface. This means that actually observing, touching, or realistically testing anything that takes place in a hydrocarbon reservoir is impossible.
Of course, some of the reservoir rock usually is brought to the surface and tested and analyzed in a laboratory to help scientists and engineers develop an understanding of the complexity of the fluid flow in the porous media deep underground. Realistic facts about such analyses, however, must not be overlooked. While hydrocarbon reservoirs have approximate volumes of hundreds of millions to tens of billions of cubic feet, the part of the hydrocarbon reservoirs rock that is brought to the laboratory for observation and testing is usually less than a few cubic feet. Furthermore, hydrocarbon reservoirs are highly heterogeneous, which means that what is analyzed in the laboratory from a few square inches of rock does not realistically represent what happens throughout the entire reservoir rock.
Therefore, while laboratory core analysis is an important and useful process of understanding the fluid flow in the hydrocarbon reservoir, it cannot realistically represent all the details and the heterogeneity throughout the tens of billions of cubic feet of hydrocarbon reservoir that is hundreds or thousands of feet under the ground. This explains the existence of assumptions, interpretations, and simplifications in the mathematical equations used to model the fluid flow in porous media. Furthermore, when the hydrocarbon reservoir is unconventional, such as the shale plays that currently are the main source of hydrocarbon production in the US, the problems become orders of magnitude more complex and even more important.
Example of AI Ethics in Production From Shale Wells
The number of assumptions, interpretations, simplifications, preconceived notions, and biases in modeling the physics of completion, hydraulic fracturing, and fluid flow in shale plays is so extensive that the mathematical modeling for completion and production optimization from shale wells becomes completely unrealistic, useless, and full of bias. This is because historical details of the physics of fluid flow in hydrocarbon reservoirs are mainly applicable to conventional plays such as sandstones and carbonates. The technology developed for conventional reservoirs has been extrapolated to unconventional reservoirs.
The mathematical equations used to model the physics of hydrocarbon production from shale wells are overwhelmed by assumptions and hardly have anything to do with facts and realities because the essence of this technology is applicable mainly to conventional reservoirs and not to unconventional reservoirs. Rarely will scientists and professional engineers (including those who have developed and are using these techniques) claim that the current mathematical modeling of hydraulic fracturing of shale wells has anything to do with reality.
This shows that using AI and ML to develop so-called hybrid models involves many assumptions, interpretations, and biases and has very little to do with the reality of using AI and ML for engineering applications. When such mathematical equations are used to generate data and then that data is combined with actual field measurements to build hybrid models, these models are forced to generate outputs and results that are predetermined by those who developed the models. This removes the actual and real characteristics of ML algorithms that are capable of modeling physics based on reality rather than on guesswork and biases. This is a good example of how AI ethics must be addressed in engineering.
When hydraulic fracturing is performed on unconventional reservoirs that are naturally fractured (such as shale), the results are quite different from what happens when hydraulic fracturing is performed on conventional reservoirs (sandstones). In shale, because of the existence of the complex natural fractures, hydraulic fracture creates a network of fractures (as shown in Figs. 1 and 2), not an elliptical hydraulic fracture (as shown in Fig. 3 for conventional reservoirs).
As shown in Figs. 1 and 2, when liquid (water) is injected in an unconventional reservoir for hydraulic fracturing, before the injection of the proppant, the formation begins to fracture. As the formation starts to fracture, the continuation of the fracture will go through the least resistant pathways in the rock. In a naturally fractured reservoir, the least resistant pathway is the network of the natural fractures while the actual fabric of the rock that has not been naturally fractured has more resistance. Hydraulic fracturing of unconventional reservoirs such as shale that are naturally fractured, therefore, creates highly complex networks of natural fractures that cannot be modeled in detail. This is because the shape, characteristics, and details of the natural fracture of the rock cannot be observed or measured throughout the reservoir. The highly complex shape of the hydraulic-fracture network in the unconventional reservoir is a function of heterogeneity and the natural-fracture network.

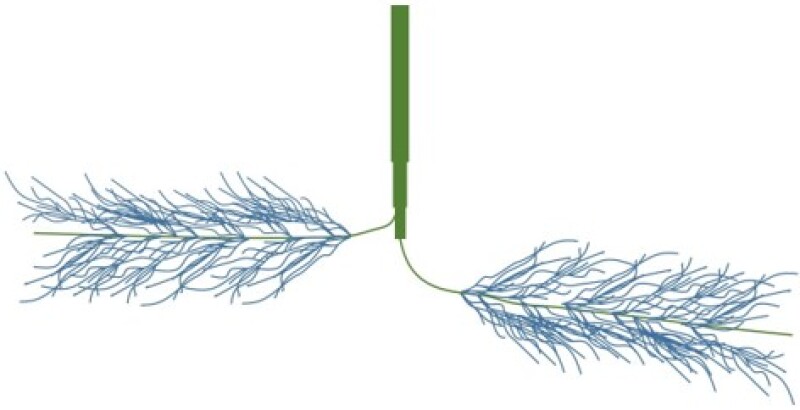
When model development of hydraulic fracturing was first performed more than 50 years ago, the shape of the hydraulic fracture in the conventional reservoir was modeled using an elliptical shape, as shown in Fig. 3. This traditional hydraulic-fracture model includes four specific characteristics that allow it to be modeled using mathematical equations. These four specific characteristics are fracture half-length, fracture height, fracture width, and fracture conductivity. Comparing the shapes of the hydraulic fractures shown in Figs. 1 and 2 with the shapes of the hydraulic fracture shown in Fig. 3 clearly reveals how different the actual shape of hydraulic fracture is between unconventional and conventional reservoirs.
When the actual hydraulic fractures look like what is shown in Figs. 1 and 2, does modeling it using the shape shown in Fig. 3 make any sense or have anything to do with reality? This is a good example of how assumptions, interpretations, preconceived notions, simplifications, and biases that are included in the mathematical equations (used to model the physics of fluid flow in porous media) are included in the hybrid models that combine them with real field measurements.
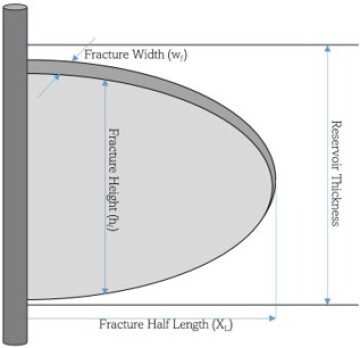
Conclusions
The ethics of AI has proven to be an important issue when AI-based models are used in decision making. AI ethics can expose biases that may have been included in the AI applications of engineering and nonengineering problem-solving. Research referenced in Part 1 of this series shows how biases such as racism and sexism in AI-based models have been exposed through AI ethics. This article shows how assumptions, interpretations, and biases developed by traditional engineers also can be included in the engineering application of AI.