This paper presents a fatigue-prediction methodology designed to extend the life of unbonded flexible risers and improve the accuracy of floating production, storage, and offloading (FPSO) vessel response analysis. The methodology combines measured-motion-response, maritime-environment, and process data to improve traditional time-domain dynamic analysis models, along with machine-learning (ML) techniques to develop a heading model for the FPSO.
Introduction
Life extension of unbonded flexible risers is a challenge because of uncertainties associated with key parameters driving deterioration, such as how the riser was designed, manufactured, and installed and how it is operated. Fatigue service life is calculated during the design phase using models based on field-specific environmental data derived for the area and loads for the required design conditions per project specifications and international standards.
Using real measured data from the riser motions and from environmental sources can reduce uncertainties significantly, improving life-extension analysis for fatigue and other failure drivers. These data, when combined with high-end engineering assessments and considerations on data reliability, provide better insight and contribute to continued operations with acceptable risk for the risers.
The complete paper presents a methodology that uses measured environmental data and FPSO and riser response data in an ML environment to build more-realistic riser-response and fatigue-prediction models.
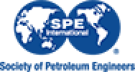