The integration of machine learning and physics-based simulation modeling offers a comprehensive approach to optimizing unconventional development and addressing parent-/child-well depletion issues. By combining the predictive capabilities of machine-learning models with the insights gained from physics-based modeling, operators can develop customized solutions tailored to the specific characteristics of the reservoir. Machine‑learning models can optimize well‑spacing, -placement, and -completion designs, while physics-based simulation modeling provides a deeper understanding of reservoir behavior and performance.
Machine learning has emerged as a powerful tool, leveraging large volumes of data to provide data-driven insights and predictive capabilities. By training machine-learning models on geologic, completion, and spacing data, operators can predict production performance and make informed decisions to enhance production and cost-efficiency.
Parent/child interactions, where production from a parent well negatively affects subsequent child wells, pose challenges in unconventional development. Benchmarking, through the comparison and analysis of performance data, plays a crucial role in understanding and mitigating these issues. By benchmarking production data, completion designs, and reservoir properties, operators can identify best practices and develop strategies to minimize the interference between parent and child wells.
By harnessing the power of data-driven insights and the knowledge gained from physics-based simulation modeling, operators can make informed decisions regarding well spacing, completion designs, and operational strategies. This integrated approach enhances decision-making, reduces uncertainties, and increases overall efficiency in unconventional development projects. As technology continues to advance, the use of machine learning and physics-based simulation modeling will further improve the optimization of unconventional development, driving the industry toward greater efficiency and sustainability.
The selected papers and suggested additional reading provide valuable insights into the evolving field of development optimization using physics-based simulation models and machine learning.
This Month’s Technical Papers
Machine Learning, Numerical Simulation Integrated To Estimate Child-Well Depletion
Machine Learning Helps Customize Spacing for Maximum Acreage Value
Study of Permian Basin Provides Insight Into Parent-/Child-Well Interactions
Recommended Additional Reading
SPE 205651 Digital Field Development Planning: A Collaboration Between Technology and Process To Enable Fast and Efficient Field Development Planning by Mohd Aminuddin Bin Md Karim, Petronas
SPE 207899 Artificial Intelligence for Infill Well Placement and Design Optimization in Multilayered/Stacked Reservoirs Under Subsurface Uncertainty by Shi Su, SLB, et al.
SPE 208529 A Comprehensive Machine-Learning Approach for Quantitatively Analyzing Development Performance and Optimization for a Heterogeneous Carbonate Reservoir in the Middle East by Ruijie Huang, Research Institute of Petroleum Exploration and Development, et al.
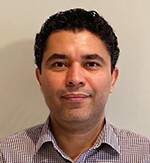
Reza Garmeh, SPE, is a reservoir engineering adviser working for Callon Petroleum. His areas of expertise include reservoir modeling and simulation studies for both conventional and unconventional resources, hydraulic fracture modeling, field development planning, data analytics, and well-performance-optimization strategies. Garmeh is also experienced in designing and modeling enhanced oil recovery projects, phase behavior, and rate transient analysis. Throughout his career, he has worked on various integrated reservoir studies and field development plan projects. Garmeh holds a PhD degree in petroleum engineering from The University of Texas at Austin and is a member of the JPT Editorial Review Board.