In the complete paper, the authors analyzed a robust, well-distributed parent/child well data set of the Delaware Basin Wolfcamp formation using a combination of available empirical data and numerical simulation outputs, which was used to develop a predictive machine-learning model (consisting of a multiple linear regression model and a simple neural network). This model has been implemented successfully in field developments to optimize child-well placement and has enabled improvements in performance predictions and net present value.
Introduction
Pervasive parent/child well pairs have complicated the development of the Delaware Basin Wolfcamp formation by introducing the need to forecast child-well performance reliably. This problem is made more difficult by the complex nature of the physical processes involved in parent/child well interactions and the variety of geometrical configurations that can be realized. In broad terms, the following three classifications of child wells can be recognized based on their spatial relationship to the associated parent well and other offset wells (Fig. 1 above):
- Type 1—Child wells completed in between two parent wells
- Type 2—Child wells completed adjacent to a parent well along with concurrently completed or codeveloped infill wells
- Type 3—Child wells completed adjacent to a single parent well
To narrow the range of complexities in the study, the authors focused on Type 2 child wells because this configuration will be used most often in future development activities and because it had the most existing field examples.
The principal objective of this assessment was to generate accurate quantitative predictions of the diminished production performance of child wells because of pre-existing parent wells.
In this work, a novel, hybrid approach is detailed involving a combination of machine-learning techniques and numerical simulations.
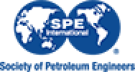