In the complete paper, machine-learning (ML) models were trained using geologic, completion, and spacing parameters to predict production across the primary developed formations in the Midland Basin. The approach of using ML to test several different combinations of spacing and completion designs can be repeated across a basin to find an economical, customized solution for each development unit.
Introduction
In contrast to conventional methods, ML offers a data-driven approach that can leverage the large amount of data generated by operators within unconventional plays. Several characteristics of ML models make them well-suited for spacing optimization, including the following:
- ML models rely on statistical methods to establish relationships between the input variables and the output variables.
- ML handles nonlinear relationships well.
- Complex variable interactions can be difficult to understand with traditional methods.
The downside is the time required to assemble the input data used for training an ML model. However, once a model is built that has an acceptable level of error along with data examples covering the range of cases to be evaluated, many alternative development scenarios can be quickly evaluated.
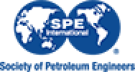