Eni and IBM developed a cognitive engine exploiting a deep-learning approach to scan documents searching for basin geology concepts, extracting information about petroleum system elements (e.g., formation name; geological age; and lithology of source rocks, reservoirs, and seals), and enabling basin geologists to perform automated queries to collect all information related to a basin of interest. The cognitive engine succeeded in identifying the correct formations, lithologies, and geological ages of the petroleum systems with an accuracy in the range of 75 to 90%.
Introduction
While commercial databases often provide summary information about basins that can be extracted easily with queries or even interactive tools, the explorationist needs to integrate such information with more up-to-date and in-depth descriptions of structural and sedimentary events occurring in the basin, descriptions that can be found only in unstructured documents. Key information about basins can be scattered across paragraphs, tables, and image captions of hundreds of technical articles, or can be embedded within pictures. Even when exploiting a traditional search engine with the name of the desired basin, the results can be unsatisfactory: first, not all the results might be relevant; second, many different variants of the basin name are often used within publications.
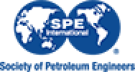