This year’s History Matching and Forecasting selections highlight innovations in surrogate modeling, artificial intelligence, and well-test analysis. These three papers leverage machine learning and hybrid methods to tackle challenges in forecasting, optimization, and reservoir characterization.
In paper SPE 220002, the authors introduce the embed-to-control observe (E2CO) framework, a deep-learning surrogate model for reservoir performance forecasting and life-cycle optimization. The E2CO model demonstrates remarkable accuracy in predicting reservoir dynamics and optimizing production under geological uncertainty, validated against the SPE10 benchmark. While currently focusing on sector-scale models, its scalable architecture supports future field-scale extensions. The integration of stochastic-gradient-based optimization enhances flexibility in handling nonlinear constraints.
Paper SPE 220876 presents a deep-neural-network-based history-matching workflow that combines a forward surrogate model for rapid multiphase-flow predictions with an inference network for parameter estimation. Its success in 2D heterogeneous reservoirs underscores its potential to streamline history matching with minimal accuracy loss. The use of synthetic data ensures controlled validation of core principles, a critical step before field deployment. Future work on extending the framework to 3D models could further solidify its applicability to complex scenarios, building on robust 2D results.
Paper SPE 221287 introduces a semi-iterative model (SIM) for well-test curve matching, improving parameter inversion accuracy in homogeneous reservoirs by leveraging key points and segments on curves. The SIM’s efficiency and precision, particularly in dual-porosity systems, set a new benchmark for automated well-test interpretation. The dependence on high-quality input data ensures reliable outcomes in controlled environments. Its success in homogeneous reservoirs paves the way for future adaptations to more‑complex challenges.
Collectively, these studies exemplify the transformative potential of machine learning and hybrid methods in reservoir management, balancing innovation with practical applicability. Their methodologies provide scalable frameworks, with current limitations serving as milestones for ongoing research rather than barriers to adoption.
Summarized Papers in This April 2025 Issue
SPE 220876 Deep-Neural-Network-Based Workflow Increases Efficacy in Solving Complex Problems by Bicheng Yan, King Abdullah University of Science and Technology, et al.
SPE 220002 Deep-Learning-Based Reservoir Surrogate Achieves Optimization Under Uncertainty by Quang Minh Nguyen, The University of Tulsa, et al.
SPE 221287 Semi-Iterative Well-Test-Matching Method Uses Featured Points To Increase Accuracy, Efficiency by Xue Guo, China University of Petroleum, et al.
Recommended Additional Reading
SPE 220790 Graph-Level Feature Embedding With Spatial/Temporal GCN Method for Interconnected Well-Production Forecasting by Ziming Xu, University of Alberta, et al.
URTeC 4033921 Application of a Sparse Hybrid Data-Driven and Physics Model in Unconventional Reservoirs for Production Forecastingby Hardikkumar Zalavadia, Texas A&M University, et al.
SPE 220995 A Hybrid Tabular-Spatial-Temporal Model With 3D Geomodel for Production Prediction in Shale Gas Formations by Muming Wang, University of Calgary, et al.
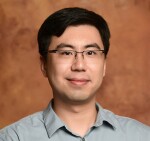
Zhenzhen Wang, SPE, is a lead research scientist and simulation engineer at Chevron Technical Center with 10 years of industry experience. His expertise spans reservoir simulation, optimization, history matching, enhanced oil recovery, and pressure transient analysis and rate transient analysis. Wang has authored more than 30 papers, reviewed more than 110 manuscripts, and serves as an Associate Editor for SPE Journal. He has contributed to program committees for the SPE Annual Technical Conference and Exhibition, Western Regional Meeting, and the Unconventional Resources Technology Conference and received the 2022 SPE Cedric K. Ferguson Medal. Wang holds a BS degree from China University of Petroleum, an MS degree from The Pennsylvania State University, and a PhD degree from Texas A&M University, all in petroleum engineering.