A tight gas field produces gas from a large heterogeneous fluvial reservoir with limited well control in margins and appraisal areas and sparse advanced logs. The principal goal of the study described in the complete paper was to provide a novel solution using machine-learning (ML) techniques to predict sandstone distribution and, to some extent, automate the process of optimizing well placement. The presented work flow overcomes low data quality, scaling, and inconsistency and builds the bridge between geoscience and artificial intelligence (AI) software platforms.
Introduction
The field is an unconventional gas reservoir covering a vast area in the Ordos Basin in China. This field produces gas from a complex and heterogeneous fluvial reservoir with limited well control for prediction of sand-body distribution.
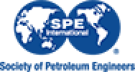