This paper discusses a project with the objective of leveraging prestack and poststack seismic data in order to reconstruct 3D images of thin, discontinuous, oil-filled packstone pay facies of the Upper and Lower Wolfcamp formation. The classification results were created by neural networks, which can be used as a substitute for traditional amplitude-vs.-offset, inversion, and cross-plotting techniques for seismic reservoir characterization.
Introduction
The problem encountered by the operator in this oil field is that the reservoir, an oil-filled packstone, is thin and laterally discontinuous. Despite having collected a high-resolution, state-of-the-art 3D seismic survey with usable frequencies up to 138 Hz, and despite having generated seismic attribute volumes in order to assist interpretation, the operator was unable to generate an interpretation manually that matched the rock-type interpretation at the wells. Therefore, the decision was taken to supplement the human interpretation with a machine-learning methodology.
Geological Setting
The East Soldier Mount (ESM) study area is approximately 200 km northeast of Midland, Texas, USA, on the eastern shelf of the Permian Basin.
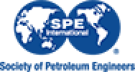