Deepwater oil and gas facilities encounter up to an estimated 5% annual production loss, estimated at billions of dollars, because of unplanned downtime. This paper describes an automated work flow that uses sensor data and machine-learning (ML) algorithms to predict and identify root causes of impending and unplanned shutdown events and provide actionable insights. A systematic application of such a method could prevent unfavorable operational situations in real time using equipment and process sensor data.
Overview
An assessment of the magnitude of deferred production resulting from unplanned shutdown from one of an operator’s deepwater facilities revealed that, overall, 43% of all shutdown events belong to unplanned-but-controllable events. Here, “controllable” means that, if the event is identified ahead of shutdown, mitigation is possible to avoid the shutdown.
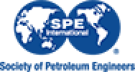