Data-driven decisions powered by machine-learning (ML) methods are increasing in popularity when optimizing field development in unconventional reservoirs. However, because well performance is affected by many factors, the challenge is to uncover trends within all the noise. By leveraging basin-level knowledge captured by big data sculpting, integrating private and public data with the use of uncertainty quantification, a process the authors describe as augmented artificial intelligence (AI) can provide quick, science-based answers for well spacing and fracturing optimization and can assess the full potential of an asset in unconventional reservoirs. A case study in the Midland Basin is detailed in the complete paper.
Introduction
Augmented AI is a process wherein ML and human expertise are coupled to improve solutions.
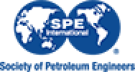