In surface-condition evaluation, visual inpection is a favored nondestructive examination (NDE) method because experienced personnel can identify defects or damages quickly. Drawbacks of this method, however, include its lack of objective quantification and description of defects. Using recent developments in machine learning (ML), image recognition, and object detection, the authors have investigated the feasibility of using ML on algorithms in recognizing objects and describing their condition.
Theory and Definitions
The authors have used the Tensorflow framework (an open source machine learning platform [www.tensorflow.org]) as a tool for ML of a convolutional neural network (CNN) for object detection without changing the original code. The purpose was to present an algorithm with images, thereby teaching it to detect objects of interest through ML. Tensorflow aids the user in the ML process with all data-management and programming steps to perform the optimization of the algorithm.
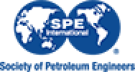