In the complete paper, the authors develop a machine-learning (ML) method to determine salt structures directly from gravity data. Based on a U-net deep neural network, the method maps the gravity downward continuation volume directly to a salt body mask volume, which is easily interpretable for an exploration geophysicist. The authors conclude that the ML-based method from gravity data complements seismic data processing and interpretation for subsurface exploration.
Introduction
In subsurface exploration, seismic is the dominant method used to reconstruct the underground image for geophysicists and geologists to locate possible hydrocarbon reservoirs. Seismic acquisition is carried out by human-induced sound waves (by airgun or vibrators) that are recorded, once reflected, on the surface.
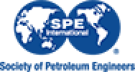