This paper discusses how machine learning by use of multiple linear regression and a neural network was used to optimize completions and well designs in the Duvernay shale. The methodology revealed solutions that could save more than $1 million per well and potentially deliver an improvement in well performance of greater than 50%. The work flow described rigorously analyzes the relationships between a significant number of well-completion variables, predicts results, and performs optimizations for ideal outcomes. The work flow is not Duvernay-specific and can be applied to other basins and formations.
Introduction
A fundamental problem for machine learning in many industries is that a responding variable is controlled not by one but by a number of predictor variables. Inferring the relationship between the responding variable and the predictor variables is of key importance.
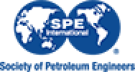