The complete paper investigates the efficient estimation of optimal design variables that maximize net present value (NPV) for life-cycle production optimization during a single-well carbon dioxide (CO2) huff ‘n’ puff (HnP) process in unconventional oil reservoirs. The NPV is calculated by a machine-learning (ML) proxy model trained to approximate the NPV that would be calculated from a reservoir simulator run. The ML proxy model can be obtained with either least-squares support vector regression (LS-SVR) or Gaussian process regression (GPR).
Introduction
The design variables considered in this study include CO2 injection rate, production bottomhole pressure (BHP), duration of the injection, and duration of production time for each cycle. To the best of the authors’ knowledge, the complete paper represents the first study that considers the life-cycle optimization problem for a hydraulically fractured well in unconventional oil formations by using two different ML-based methods.
Modeling and Sensitivity Analysis
Reservoir and Fluid Model.
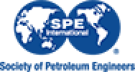