Avid readers of recent technical literature in upstream energy can identify an upward trend in the frequency of studies that use large-data-set analysis to improve decision-making regarding production and operations. While big data and artificial intelligence had originally been fascinating concepts without wide examples of practical use, recent studies have showcased more practical uses of these methods of data analytics.
The technical arena of oilfield chemistry, both that of the natural downhole environment and that of chemistry introduced downhole, provides a rich testing ground for advanced uses of data. Whereas early uses of data in the upstream chemical space included use of design of experimentation for vetting or developing new chemistries, today data analytics enables, for example, production allocation based on assessment of produced hydrocarbons. Featured studies showcase this type of application of data analytics to improve production assessment. One example reviewed various geochemical methods to allocate zonal contribution to production by analyzing produced fluid composition and decoupling the contributions of individual zones’ fluids. Another selection measured the carbon isotope signatures of gas from individual zones and used it to determine the thermal maturity of the gases from different reservoirs; this work has the potential for similar zonal allocation assessment of gas production.
In its current maturity, machine learning also provides significant potential to improve our understanding and prediction of downhole chemical reactions. In another featured study, a geochemical data set of staggering proportions was populated with water composition data from many years and wells. Coupled with the equations that govern many downhole reactions to use machine learning, the presented tool allowed improved modeling and prediction of, for example, inorganic scaling behavior under downhole conditions.
This Month’s Technical Papers
Geochemical Approaches Assist Production Allocation of Commingled Fluids
Chemical and Carbon Isotope Composition Proves Effective as Gas Diagnostic Tool
Reduced-Order Models Blend Chemistry, Machine Learning for Water-Property Analysis
Recommended Additional Reading
SPE 206091 Asphaltenes: Fundamental Principles to Oilfield Applications by Oliver Mullins, SLB, et al.
SPE 211238 Detection and Monitoring of Corrosive Oilfield Microorganisms Through Novel Biomarker Technologies by Sven Lahme, ExxonMobil, et al.
SPE 207340 Toward the Rational Design of Chemical Formulations for EOR From Carbonates: Molecular-Level Understanding of Carbonate Wettability and Its Reversal by Surfactants and Ions by Shixun Bai, University of Wyoming, et al.
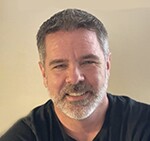
Michael Fuller, SPE, is a senior completions subject-matter expert in the Chevron Technical Center, where he works in materials, fluids, and methods for completions, drilling, and stimulation operations as a part of Chevron’s Wells team. In his 19 years working in global roles in upstream oil and gas, he has been issued several patents and produced numerous industry publications. Fuller holds a PhD degree in chemistry from Northwestern University and a BS degree in business chemistry from Northern Illinois University. He is a member of the Chemical Science Roundtable at the National Academy of Science, Engineering, and Medicine and was vice-chair for the 2023 SPE International Conference on Oilfield Chemistry.