The complete paper discusses Equinor’s operation planning tool (OPT), developed to present planners with the technical conditions of a platform, identify potentially dangerous combinations of concurrent activities, and propose learnings from 8 years of incident recordings. The OPT provides a single interface detailing a plant’s technical conditions, all planned work orders, and relevant lessons from previous incidents. By reducing reliance on personal experience, the tool has improved risk identification and handling, achieved faster knowledge transfer to new employees, and focused cross-platform knowledge sharing.
Introduction
The operator’s requirement that all incidents or near-misses be reported has resulted in a database of over 108,000 incidents. Alongside lessons learned from previous incidents, planners must consider the suitability of concurrent jobs and any technical status that may affect the safety of executing a planned job at a specific time.
Before 2018, planners had to manually consult eight data sources, each with a separate user interface (UI), to obtain an overview of the plant’s technical status, concurrent jobs planned, and relevant lessons learned from previous incidents.
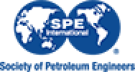