The complete paper proposes a data-driven proxy model to effectively forecast the production of horizontal wells with complex fracture networks in shales. With a multilayer gated recurrent unit (GRU) cell, the proxy model is coupled with newly developed deep-learning methods, an attention mechanism (Att-GRU), skip connection, and cross-validation to deal with time-series-analysis (TSA) issues of multivariate operating and physical parameters. Results indicate that the Att-GRU method can forecast the production for shale gas wells with complex fracture networks accurately at a given time and with variable bottomhole pressure (BHP) while maintaining high calculation efficiency.
Methodology
The authors write that their main interest is to develop a physics-based data-driven model to speed up the simulation of complex fracture networks in shale reservoirs. Results show that the deep-learning model can faithfully recapitulate the variable BHP condition in all of its intricacies with multivariate physical input.
In this section of the complete paper, the involved physical background and the corresponding governing equations are clarified.
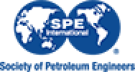