The trading of technologies is nothing new for the upstream oil and gas and medical communities. The connection makes sense, particularly since both disciplines rely heavily on applied math for diagnoses. It should come as no surprise, then, that a popular paper at the 2021 Virtual SPE/IADC International Drilling Conference proposed a medically inspired approach to prevent catastrophic drilling system failures brought on by downhole shocks (SPE/IADC 204098).
The paper describes a “drilling electrocardiogram” that diagnoses “arrhythmic drilling” similarly to how medical electrocardiograms diagnose dangerous vibration anomalies in heart patients.
The approach classifies shock waveforms acquired at 31,250 Hz downhole. The shock signals are treated as drilling electrocardiograms (D-ECG) that are processed using clustering algorithms and merged with drilling incidents to identify in real time an arrhythmic signature pattern that can lead to catastrophic failures.
A Revelation
Justo Matheus, senior control engineer for Schlumberger and lead author of the paper, said that, in studying field incidents in which rotary steerable system (RSS) bottomhole assemblies (BHA) had been severely damaged by shocks, the signature patterns reminded him of ECGs (Fig. 1).
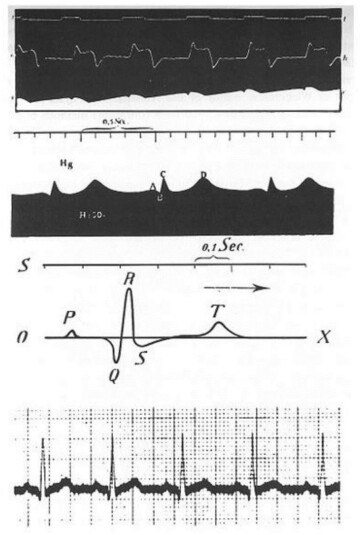
This led him to medical libraries, which in turn led to a revelation—that throughout the 3 decades that downhole vibration measurements while drilling (MWD) have been studied, only the analysis of the amplitude and root mean square values of shocks had been the focus.
No one had considered frequency for diagnosing downhole shocks. It was this revelation that drove the concept of the D-ECG based on shock waveforms acquired at high frequency in real time to prevent failures of the BHA.
What Is Normal and What Is Not?
Drilling-generated shocks and vibrations affect rate of penetration, directional control, and wellbore quality, making them among the main causes of failures in drilling.
“Shocks are present almost all the time,” Matheus said. “The challenge is in knowing which are normal and which are not.”
RSSs are equipped with measurement devices such as magnetometers, accelerometers, and shock and vibration sensors that obtain statistical information from which whirl, bit bounce, and stick/slip severity are inferred. Often, however, the derived statistics are not sufficient to distinguish between normal drilling vs. abnormal drilling for a location in the wellbore.
Recent electronic advances enabled the development of high-resolution drilling dynamic data recorders, extending the sampling frequency from traditional 100 Hz to 1,600 Hz. However, most of these devices are for data recording only. There is no real-time communication with surface and no capability to inform about drilling conditions downhole.
RSSs are also equipped with sophisticated navigation sensors, similar to MWD tools and capable of recording signals in fractions of a second. To acquire shock waveform data downhole during drilling operations, a shock frequency module is programmed in the RSS electronics hardware using a field-programmable gate array and is run inside the control unit. The shock acquisition and processing are performed at 31,250 Hz—300 times the current standard processing-sampling frequency.
“Using machine learning, we were able to perform a frequency analysis to recognize abnormal shock signatures,” Matheus said. “We discovered two destructing shock patterns and a new frequency pattern, which we added as a signature of dangerous drilling conditions, or arrhythmic drilling.”
Using the D-ECG methodology, historical shock events have been acquired and analyzed using machine-learning algorithms to estimate abnormal downhole situations such as whirl, bit bounce, and formation changes, with the potential to apply them in real time to improve trajectory control and prevent catastrophic drilling failures.
Introducing a Drilling ECG
The RSS electronics board records shock waveforms by event. Whenever a shock is above a certain threshold, the electronics board will record 2,048 samples at 31,250 Hz. These waveforms can be transformed from time domain to frequency domain to create a shock spectrogram that represents the signal amplitude in a specific frequency at a timestep.
Earlier models, including using deep neural network architecture to detect simulated drilling conditions downhole, were very challenging to implement inside the RSS electronics board, so a plan was devised to find a less-complex solution to classify the downhole shocks in real time. The shock data acquired in time domain are transformed to frequency domain using a fast-Fourier-transform signal, which represents the D-ECG. Then the D-ECGs are grouped using the K-means algorithm, an unsupervised clustering algorithm often used in machine learning.
Several data sets comprising high-frequency shock data were correlated with field report incidents. Because the objective was to identify D-ECGs that might lead to a failure, the focus was exclusively on identifying “good" and "destructive" drilling conditions. Good drilling means that the RSS performed as planned and field maintenance did not find any damaged hardware.
Destructive drilling condition means that the RSS was unable to perform the job and the maintenance facility found damaged components while servicing the tool. The destructive signature can be downloaded to the RSS tool configuration for identifying hazardous or abnormal drilling conditions in real time.
Reading the Results
Fig. 2 shows a normal D-ECG. If the RSS is suffering this type of shock, there is a very low probability of a failure downhole and the tool will most likely finish the job.
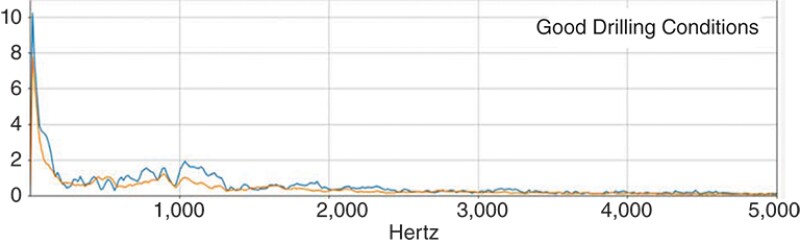
Fig. 3 shows the three types of D-ECGs that have a high probability of creating a failure downhole. Data have shown that, if an RSS experiences downhole shocks with any of these signatures, the tool is likely to have a failure downhole.
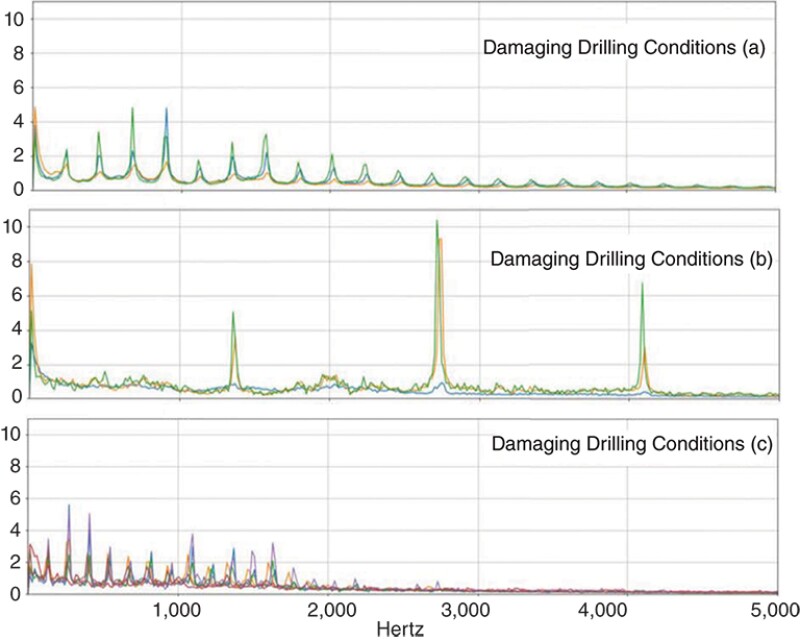
Creating an RSS Health Framework
Using a health informatics framework, any member of a cardiac patient’s team of physicians and nurses can easily search for and retrieve diagnostic images to collaborate on treating the patient. A similar framework has been created to treat and maintain RSSs across the world. The RSS health framework keeps track of the electronic boards and collars, applying machine-learning algorithms to predict the remaining useful life of the assets.
According to Matheus, data coming from different sources such as RSS downhole data, surface data, field incidents, and maintenance systems are merged into health-analyzer repositories that can be deployed in the rig-control systems or downhole.
The arrhythmic drilling signatures that are identified can be deployed downhole easily to transmit the operational state from downhole to surface in real time. Then, control strategies can be implemented to manipulate surface actuators such as topdrives, drawworks, and mud pumps.
Matheus believes the methodology can be extended to create models to recognize several drilling conditions for improving drilling efficiency.
“The framework created to treat and maintain downhole tools across the world has enabled a democratization of the health data recorded inside the downhole tools that will allow engineers, tool subject-matter experts, and data scientists from different backgrounds to collaborate to create solutions to extend the reliability of drilling systems and optimize the well-construction process,” he said.
For Further Reading
SPE/IADC 204098 A Medical-Inspired Framework To Classify Downhole Shocks Waveforms While Drilling by J. Matheus, M. Ignova, and D. Amaya, Schlumberger
SPE 194952 Recognizing Abnormal Shock Signatures During Drilling With Help of Machine Learning by M. Ignova, J. Matheus, D. Amaya, et al., Schlumberger