Description of the Technology
Models are needed to develop and operate petroleum reservoirs efficiently. Data-driven reservoir modeling [(also known as top-down modeling (TDM)] is an alternative or a complement to numerical simulation. TDM uses the so-called “big-data” solution (machine learning and data mining) to develop (train, calibrate, and validate) full-field reservoir models on the basis of measurements rather than solutions of governing equations.
Unlike other empirical technologies that forecast production, or only use production or injection data for its analysis, TDM integrates all available field measurements (well locations and trajectories, completions, stimulations, well logs, core data, well tests, seismic, and production/injection history—e.g., choke settings) into a full-field reservoir model by use of artificial-intelligence technologies. Intelligent Solutions, as the inventor of TDM, has recently released software application “IMagine” for TDM development.
TDM is a full-field model wherein production [including gas/oil ratio (GOR) and water cut] is conditioned to all measured reservoir characteristics and operational constraints.
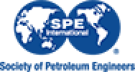