Current theoretical formulations of assisted-history-matching (AHM) problems within the Bayesian framework [e.g., ensemble-Kalman-filter (EnKF) and randomized-maximum-likelihood (RML) problems] are typically based on the assumption that simulation models can reproduce field data accurately within the measurement error. However, this assumption does not hold for AHM problems of real assets. This paper critically investigates the impact of using realistic, inaccurate simulation models. In particular, it demonstrates the risk of underestimating uncertainty when conditioning real-life models to large numbers of field data.
Introduction
Improved simulation and history-matching techniques have still not cured the chronic ailment of systematically underestimating uncertainty in forecast results.
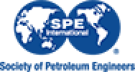