In the authors’ study, a machine-learning predictive model—boosted decision tree regression (BDTR)—is trained, tested, and evaluated in predicting liquid holdup (HL) in multiphase flows in oil and gas wells. Results show that the proposed BDTR model outperforms the best empirical correlations and the fuzzy-logic model used in estimating HL in gas/liquid multiphase flows. Using the BDTR model with its interpretable representation, the heuristic feature importance of the input features used in building the model can be determined clearly.
Introduction
Machine-learning approaches in predicting HL in multiphase flows have been recently studied to improve prediction accuracy compared with existing empirical correlations. However, these approaches ignore the heuristic feature importance of the input parameters to the predicted HL values.
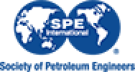