Assessing fatigue damage in marine risers caused by vortex-induced vibrations (VIV) serves as a comprehensive example of using machine-learning methods to derive assessment models of complex systems. A complete characterization of the response of such complex systems usually is unavailable despite massive experimental data and computation results. These algorithms can use multifidelity data sets from multiple sources. In the complete paper, the authors develop a three-pronged approach to demonstrate how tools in machine learning are used to develop data-driven models that can be used for accurate and efficient fatigue-damage predictions for marine risers subject to VIV.
Introduction
In this study, machine-learning tools are developed to construct a digital twin of a marine riser.
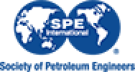