Formation evaluation stands as a cornerstone in determining the potential of a wellbore in the exploration, development, and production of oil and gas resources. Using a suite of tools such as well logging, core analysis, formation testing, and well-testing analysis, formation evaluation provides critical insights into reservoir properties. For instance, well-test analysis enables reservoir-parameter inversions, well-productivity evaluation, and reservoir-boundaries identification for both conventional and unconventional reservoirs.
The inherent subjectivity of human interpretation, however, can introduce challenges, often resulting in nonunique solutions during certain formation evaluation processes. This subjectivity can lead to inefficiencies and inaccuracies in predictions or estimations. Fortunately, recent advancements in computing power and artificial-intelligence (AI) algorithms have catalyzed a paradigm shift within the field. Machine-learning techniques, renowned for their robust nonlinear regression capabilities, are being increasingly explored for formation evaluation, offering the potential for enhanced accuracy and efficiency. Consequently, the integration of AI into formation evaluation workflows has garnered significant attention.
The papers featured here highlight the practical applications of AI in formation evaluation. In paper IPTC 23411, a case study combines rock typing and machine-learning neural-network techniques to predict permeability in heterogeneous carbonate formations in an Abu Dhabi offshore field. Shifting focus to southern Iraq, paper SPE 218890 evaluates various imputation techniques for predicting missing core permeability and porosity in a carbonate reservoir, demonstrating the adaptability of AI across diverse geological settings. Furthermore, a case study in paper IPTC 23381 from Northern Colombia demonstrates the efficacy of AI in determining electrical properties by integrating log data, rock types, facies, and digital core analyses.
The integration of AI within the oil and gas industry is rapidly gaining momentum. With ongoing advancements, the future of formation evaluation promises transformative changes, leading to more-efficient and accurate reservoir characterization methodologies.
This Month’s Technical Papers
Integrated Technique Predicts Permeability Accurately in Carbonate Reservoirs
Machine Learning Aids Imputation of Missing Petrophysical Data in Iraqi Reservoir
Machine Learning Helps Predict Electrical Properties of Heterogeneous Reservoirs
Recommended Additional Reading
SPE 218881 Advancing Reservoir Understanding: Integrating Deep Learning Into Automated Formation Microimaging Log Interpretation for Enhanced Reservoir Characterization and Management by Amr Gharieb, Khalda Petroleum, et al.
SPE 218859 Formation Evaluation and Behind-Casing Opportunity Analysis Using Multioutput Regression and Machine-Learning Techniques by H. Hassani, RiseHill Energy Solution, et al.
IPTC 23372 Decoding the Stratigraphic Heterogeneity of Bengal Basin, India, Using Supervised Machine Learning—A Case Studyby Arijit Sahu, Oil and Natural Gas Corporation, et al.
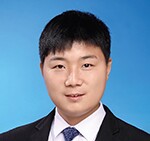
Zhiming Chen, SPE, is a professor of petroleum engineering at the China University of Petroleum-Beijing. He holds a BS degree from Yangtze University and MS and PhD degrees from the China University of Petroleum-Beijing. In 2016–17, Chen was a visiting scholar at the Department of Petroleum and Geosystems Engineering at The University of Texas at Austin. His interests are well testing, numerical simulation, and CO2 sequestration, focusing on the development of unconventional reservoirs with multistage hydraulic fractures. Chen has authored or co-authored more than 30 technical peer‑viewed publications. He is a member of the Cedric K. Ferguson Medal Committee and the Reservoir Award Committee. Chen has served as associate editor for SPE Journal.