The complete paper explores the use of multilevel derivative-free optimization for history matching, with model properties described using principal component analysis (PCA) -based parameterization techniques. The parameterizations applied are optimization-based PCA (O-PCA) and convolutional-neural-network-based PCA (CNN-PCA). Mesh adaptive direct search (MADS), a pattern search method that parallelizes naturally, is used for the optimizations required to generate posterior models. The use of PCA-based parameterization reduces considerably the number of variables that must be determined during history matching, but the optimization problem can still be computationally demanding. The multilevel strategy addresses this issue by reducing the number of simulations that must be performed at each MADS iteration.
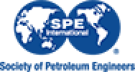