A field-development plan consists of a sequence of decisions. Each action taken affects the reservoir and conditions any future decision. The presence of uncertainty associated with this process, however, is undeniable. The novelty of the approach proposed by the authors in the complete paper is the consideration of the sequential nature of the decisions through the framework of dynamic programming (DP) and reinforcement learning (RL). This methodology allows moving the focus from a static field-development plan optimization to a more-dynamic framework that the authors call field-development policy optimization.
×
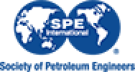
Continue Reading with SPE Membership
SPE Members: Please sign in at the top of the page for access to this member-exclusive content. If you are not a member and you find JPT content valuable, we encourage you to become a part of the SPE member community to gain full access.