In reviewing the long list of papers this year, it has become apparent to me that the hot topic in reservoir simulation these days is the application of data analytics or machine learning to numerical simulation and with it quite often the promise of data-driven work flows—code for needing to think about the physics less.
Data-driven work flows have their place, especially when we have a lot of data and the system is very complex. I’m thinking shales especially, but seeing it being applied to more conventional reservoirs gave me a moment of pause.
I can’t help but think that, in terms of the hype cycle as related to the application of machine learning to numerical simulation, we may be approaching the peak of inflated expectations. I say “approaching,” because many companies appear to be dipping their toes in the water, perhaps because they think they should, but few are truly committing to it. Many vocal champions of the approach exist, but most decision-makers just don’t understand it yet.
If we cannot explain how something works simply, then thoughtful leaders will tend not to trust it. Whether it be numerical-simulation findings or self-organizing neural networks, the need will always exist for a deep understanding and clarity of explanation of both the discipline and method used. To decision-makers, it will be an attractive concept, but they will generally ask to validate against more traditional methods.
I look forward to a future when we are through the trough of disillusionment and start climbing the slope of enlightenment to a new level of productivity. I suspect, though, that it will take at least another 5 years, as our current crop of knowledgeable evangelists become decision-makers themselves and can put in place work flows and teams to leverage the approach appropriately for their problems, intelligently leveraging their years of hard-won experience.
I will lay a wager with you, though, that when that time comes, those new ways of working more efficiently will rely just as much if not more upon a deep understanding of reservoir engineering as our current methods.
I hope you enjoy these papers, which include examples of both the new approach as well as tried-and-true approaches.
This Month’s Technical Papers
Reservoir Simulation, Modeling of a Complex Offshore Field Uses AI and Machine Learning
Deep Sequential Surrogate Model Enables New Work Flows
Reservoir Characterization, Scenario-Based Models Optimize Development Planning
Recommended Additional Reading
SPE 202436 Fast Modeling of Gas Reservoirs Using Proper Orthogonal Decomposition/Radial Basis Function (POD/RBF) Nonintrusive Reduced-Order Modeling by Jemimah-Sandra Samuel, Imperial College London, et al.
IPTC 21417 A New Methodology for Calculating Wellbore Shut-In Pressure in Numerical Reservoir Simulations by Babatope Kayode, Saudi Aramco, et al.
SPE 201658 Mechanistic Model Validation of Decline Curve Analysis for Unconventional Reservoirs by Mikhail Gorditsa, Texas A&M University, et al.
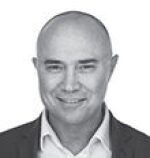
Mark Burgoyne, SPE, is a principal reservoir engineer for Santos. He has more than 26 years of industry experience, including technical leadership and subsurface management roles with Santos and hydraulic fracturing, cementing, and coiled tubing roles with Schlumberger. Burgoyne holds a bachelor’s degree in chemical engineering, a master’s degree in petroleum engineering, and an MBA degree.