Despite considerable progress in the development of rapid evaluation methods for physics-based reservoir simulators, a significant gap still exists in the acceleration and accuracy needed to enable complex optimization methods. The complete paper describes an end-to-end deep surrogate model capable of modeling field and individual-well production rates given arbitrary sequences of actions (schedules), including varying well locations, controls, and completions. Results provide concrete measures of the efficacy of the deep surrogate model as an enabling technology for the development of optimization techniques previously out of reach because of computational complexity.
Introduction
Recent advances in machine learning, particularly in deep learning, have enabled breakthroughs in technically challenging areas such as computer vision, natural language processing, and speech processing. The authors expand on these powerful algorithms to develop a deep neural network (DNN) surrogate model for reservoir dynamics.
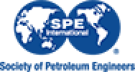