If you talk to a typical subsurface professional working on unconventionals today (e.g., a reservoir engineer, completion engineer, geologist, petrophysicist, etc.) as I have in person and through media such as LinkedIn, you will find that many lament one key thing: Our sophisticated models have been reduced too much. Of course, I am generalizing and those are not the words they use; the lamentations come in many forms.
The dissatisfaction with oversimplification is most easily observed as distaste for the type curve, the simplified model we use to predict upcoming new drills. (Yes, I know many of you will want to refer to them by their ”proper” name: type well curve; I will be sticking with the colloquial version.) A simple meme posted on LinkedIn about type curves garnered one of the most engaged conversations I have seen amongst technical staff. The responses varied from something like “Thank God someone finally said this out loud” to comments such as “I don’t know anything better than type curves.”
Most comments were closer to the former than the latter.
What is even more remarkable is that our investors feel the same. In personal conversations, many of them refer to our type curves simply as “lies.” This perception, coupled with the historical lack of corporate returns, led investors away from our industry in droves. Many within the industry see it differently and want to blame the exodus on other factors such as oil and gas prices, climate change, competition from renewables, other environmental, social, and governance (ESG) issues, the pandemic, or OPEC’s unwillingness to “hold the bag” any longer.
If you ask them, though, investors will tell you a simple answer: The unconventional business destroyed way too much capital and lied too much through the type curves.
Why is it that both investors and technical staff are unhappy with our ability to accurately model future performance? Why can’t we deliver returns?
The typical unconventional-focused oil and gas company has two models that are critical to the business. First is the subsurface model, with which we are all intimately familiar in its various forms, and the second is the corporate financial model, which is focused on cash flows, income, and assets/liabilities.
It is unfortunate that the two models are separate. It means we must simplify one or both so they can communicate with each other.
How can you observe this oversimplification while it is happening? It is happening when the finance staff say, “Please just give me a simple type curve and well count; I need to model, optimize, and account for debt/leverage, equity, and cash flows.” Meanwhile, the technical staff say, “Please just give me a CAPEX budget or a well count; I need to model, optimize, and account for well spacing, completion design, land constraints, and operational constraints.”
Looking back, we know that the winner in this tug-of-war of competing needs was the type curve.
Enter the DIY Type Curve
The financialization of unconventionals (as observed through large equity raises and vast amounts of accumulated debt) meant that the financial model was more important. As a result, the subsurface model was simplified as much as was possible and the type curve became the industry’s standard tool. No doubt helping to sustain this status is the fact that type curves are easy to build. They also allow for ample creative license for people to make them what they want to be, and financial professionals have understood this well enough to make their own.
Today, anyone with access to off-the-shelf tools and a little know-how can reasonably create a set of type curves across the entire North American unconventional concept in a day or two. Maybe not manually, but certainly programmatically.
As easily as type curves are created, they can be manipulated and biased. Most are familiar with survivorship bias as it exists in type curves (i.e., the tail tends to be dominated by long-lived wells, while the dead wells disappear), but there are many other ways bias creeps in.
Some examples of bias include:
- Assuming lateral length degradation does not exist and/or is linear (by normalizing production to per foot or a specific lateral length).
- Selection bias through filtering out low performers. ("Surely, we are smart enough to avoid that train wreck.")
- Univariate extrapolation for unobserved scenarios such as larger completions and/or tighter well spacing.
As a result, our investors, now able to make their own type curves, presented a difficult challenge to the industry. When they showed up to our investor presentations, the aforementioned type curve biases were increasingly being interpreted as outright lies. This fueled the desire of investors to build their own. Eventually, a cottage industry would rise around this idea, owing its existence to the sudden demand for independent type curves.
All of this might have been acceptable had we, the unconventional sector, been generating returns for our investors. Unfortunately, only those who entered, pumped up value, and promptly sold, delivered returns to their investors. Companies that developed their assets were not making money.
Imagine for a second you are an investor. The type curves are showing incredible returns, yet the company is not making tons of money. Type curves are simple enough, you think, which means the next step is to go make your own. In doing so, you are likely to reach a different answer that is less optimistic. Wouldn’t you then feel deceived?
Many subsurface teams will retort, “Just make a better type curve.” Theoretically, this is feasible. But upon investigation, it loses appeal.
The teams who are making “better type curves” usually just end up making more of them. One example is a company that has more than 100 different type curves for a single asset. Communicating 100 type curves to the finance team and the investment community is not very practical. So, in effect, that gets narrowed down to a few possibilities and, thus, the firm does not get the chance to understand the full range of possibilities.
Not Just Type Curves
Since about 2014, almost every operator of an unconventional play has tried to push the boundaries for tighter well spacing. Originally, it was just tighter horizontal well spacing. Then it became “The Wine Rack,” followed up by “The Cube,” “Dominator,” et al. We will keep things simple by using the terms “cube” or “cubing,” which proved to be extremely popular for years in industry. Most of that time, up until 2018, investors were neutral on the matter (Fig. 1). But by late 2018, investors finally soured and started to head for the exits.
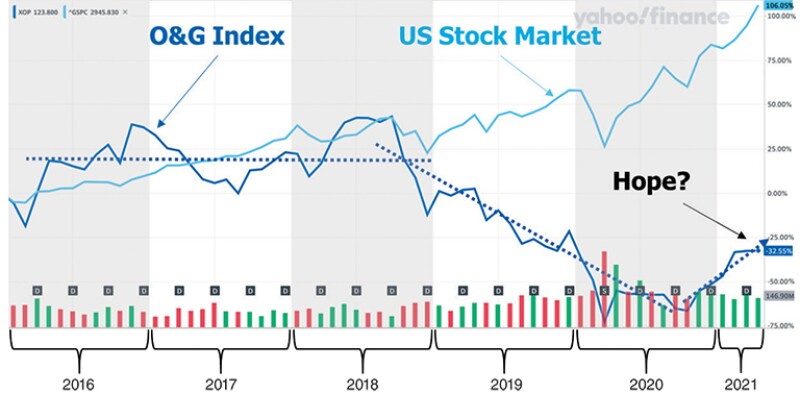
In our industry, it is common for professionals to blame external macro factors such as the cyclical nature of commodity pricing, increasing renewable energy competition, other ESG factors, or regulatory scrutiny.
However, a simpler explanation exists: Our investors were not making money because we were not.
After years of flat stock prices and no improvement in company performance on the horizon, cubing was received poorly once the results came in. Ever since, the industry has been on a downward trend. So much so that I would argue COVID-19 was just a blip.
Recent oil and gas price gains have given the industry reason to be tentatively optimistic again, but investors do not seem convinced yet.
What went wrong with cubing? The root still lies in the need to simplify either the corporate financial model or the subsurface model. What is interesting about spacing decisions, though, is that the corporate financial model gets simplified (since spacing is up to the subsurface team) and is only present as a net present value (NPV) based on an assumption of weighted average cost of capital (WACC), which is usually 10% or some higher cutoff to account for risk. This link provides an explanation of WACC https://www.investopedia.com/terms/w/wacc.asp.
There is an easy way to gain intuition around what is happening in the simplified model (Fig. 2). In general, a single well’s highest NPV is at the greatest well spacing, while its lowest NPV is at the tightest well spacing.
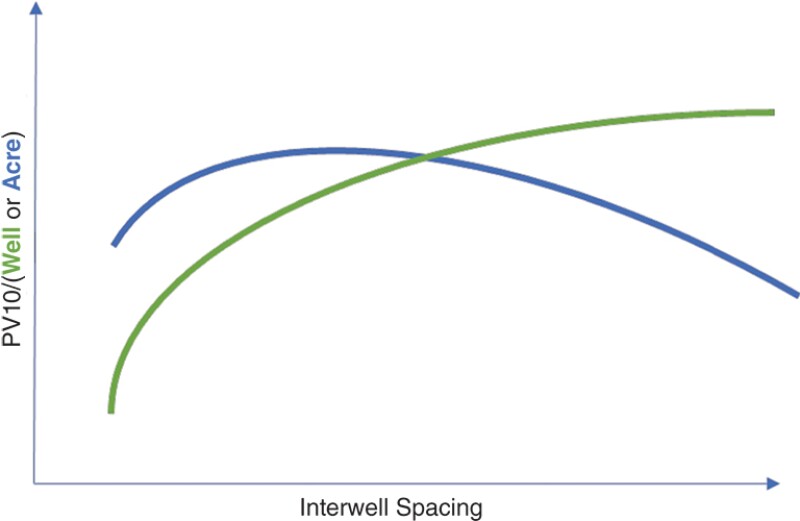
The problem, though, is that companies are not just drilling single wells; they need to optimize spacing across pads, units, acreage positions, and across the portfolio. The highest NPV per acre/pad/unit/asset/basin is found toward the tighter end of well spacing which is what led to cubing.
Another unfortunate aspect to this approach is that the subsurface model also gets simplified.
Few pay attention to the interactions among well spacing, completion design, and geology. Type curves are too unwieldly to really handle the complexity, and simulation is often too intensive. It is intuitive to think that, at tighter well spacings, well performance is influenced to a greater degree by larger completion designs and vice versa.
At a minimum, it would take a very large number of type curves. To accurately model the numerous outcomes would require around 1 million scenarios per potential location. Because of this, teams tend to pick their completion and spacing design, then optimize by looking at small offset steps in either direction (e.g., wider/tighter spacing or larger/smaller job size). A better approach would be to model all the possible outcomes and see what makes the most sense.
The real issue with the typical spacing approach is that it assumes the corporate financial performance is best served by building out the highest number of economic locations (also known as maximizing NPV). Capital is finite and sometimes it will take so long to get to the additional locations that a company ends up degrading overall value by adding them.
This simplification also does not account for how corporate cash flows respond to the changes in the development plan. Nor does it account for a company’s ability to raise and pay back debt and equity. In short, ramifications to corporate returns are not well understood and are thus adversely affected.
Stop the Oversimplification
I propose a thought experiment: Even if prices are flat into perpetuity, should optimized well spacing and design stay static in perpetuity, regardless of changes in cash flows and the ability to raise debt/equity?
I think it is time to start thinking about what the better version of an E&P company looks like.
Price can bail out bad behavior, as it appears it will now, but price runups are always temporary. The company that will win back investors in the long run will be the company that understands how to optimize development planning within the context of corporate returns. In order to do that we must stop simplifying the subsurface and corporate financial models just for communication. Both are at some level just math.
Why not combine them?
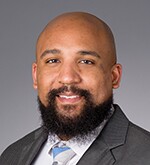
Justin Hayes joined Bedrock Energy Partners in October 2018 to lead reserve analysis, subsurface evaluation, and development planning and optimization efforts. Prior to joining Bedrock Energy Partners, he was with Anadarko Petroleum Corporation (APC) where he managed reservoir engineering and analytics efforts for APC’s Powder River Basin (PRB) asset. In the PRB, Hayes led analytical- and physics-based efforts for optimizing PRB’s appraisal and development planning. Prior to that, he was the subject matter expert on subsurface and economic evaluations for unconventional assets on APC’s Advanced Analytics and Emerging Technology team where he led efforts to develop analytical- and physics-based techniques and tools for deployment in asset development teams, acquisition and divestiture efforts, US onshore exploration, and companywide portfolio optimization. Previously, Hayes worked in various roles including development and operations for US onshore and deepwater Gulf of Mexico.
He holds a BS degree in petroleum engineering from the Colorado School of Mines, where he was a Boettcher Scholar, and an MBA from the Jones Graduate School of Business at Rice University. Hayes serves as President and Chairman of the board of directors for the nonprofit Harbor School Houston, an early-childhood education center for at-risk students.