The authors of the complete paper propose a novel approach to data-driven modeling of transient production of oil wells, applying transformer-based neural networks trained on multivariate time series composed of various parameters of oil wells measured during exploitation. By tuning machine-learning models for a single well (ignoring the effect of neighboring wells) on open-source field data sets, the authors of the paper demonstrate that the transformer-based method outperforms recurrent neural networks (RNNs) with long- and short-term memory (LSTM) and gated recurrent-unit cells in the forecasting of bottomhole pressure dynamics.
Introduction
The authors apply a novel deep-learning algorithm called a transformer to build surrogate models for simulations of well performance. Transformer architecture initially was developed for natural-language processing problems. However, in recent years, researchers have adapted transformers for time-series forecasting.
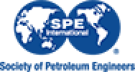