Maximizing stimulated natural and hydraulic-fracture networks is a primary concern for economic production from a horizontal shale gas well. Geomechanical facies and preexisting fractures in each stage are identified based on similarities in formation characteristics. This often requires analyzing large volumes of drilling, logging-while-drilling (LWD), and measurement-while-drilling (MWD) data. In the complete paper, the authors develop a methodology that calculates mechanical specific energy (MSE) using real-time drillstring acceleration signals.
Introduction
The Marcellus Shale Energy and Environmental Laboratory (MSEEL) aimed to improve stimulation efficiency by collecting, integrating, and analyzing real-time LWD and MWD data.
×
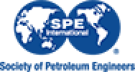
Continue Reading with SPE Membership
SPE Members: Please sign in at the top of the page for access to this member-exclusive content. If you are not a member and you find JPT content valuable, we encourage you to become a part of the SPE member community to gain full access.