Across the exploration-and-production (E&P) industry, several projects were found to underperform compared to the promises made at the time of project approval. Investment decisions on upstream projects rely, to a large extent, on the robustness of the predicted ultimate recovery and production forecast associated with the chosen development concept. The challenge for the E&P industry is to ensure that project approvals are based on realistic forecasts. This paper is intended to increase awareness among the forecasters and the decision makers about pitfalls associated with production forecasting.
Introduction
Recently, an industry benchmarking consortium concluded the sixth of a series of long-term production-attainment studies, which reviewed the production profiles of 59 major development projects from different hydrocarbon-producing regions. For the majority of projects, the forecasted production was not achieved.
Long-term production forecasts drive company strategies, portfolio choices, sales contracts, and shareholder promises. Too-optimistic forecasts result in underdelivery of projects, with often far-reaching consequences. Too-pessimistic forecasts, on the other hand, may lead to undervaluation of assets.
The value of an E&P company is determined by the projects in its portfolio. Some projects in the portfolio are mature, with a high likelihood of progressing to the execution phase. Some projects are immature, with a possibility of not reaching the execution stage (Fig. 1). For each project, it is important to have a realistic expectation of costs and production because this is the basis for business decisions. In addition, for immature projects, it is equally important to estimate the likelihood that they will be executed. At the portfolio level, this probability of maturation will lead to an appropriate expectation of expenditure and production and will facilitate the making of higher-level business decisions. Fig. 2 shows how to combine realistic forecasting at the individual-project level and at the portfolio level.
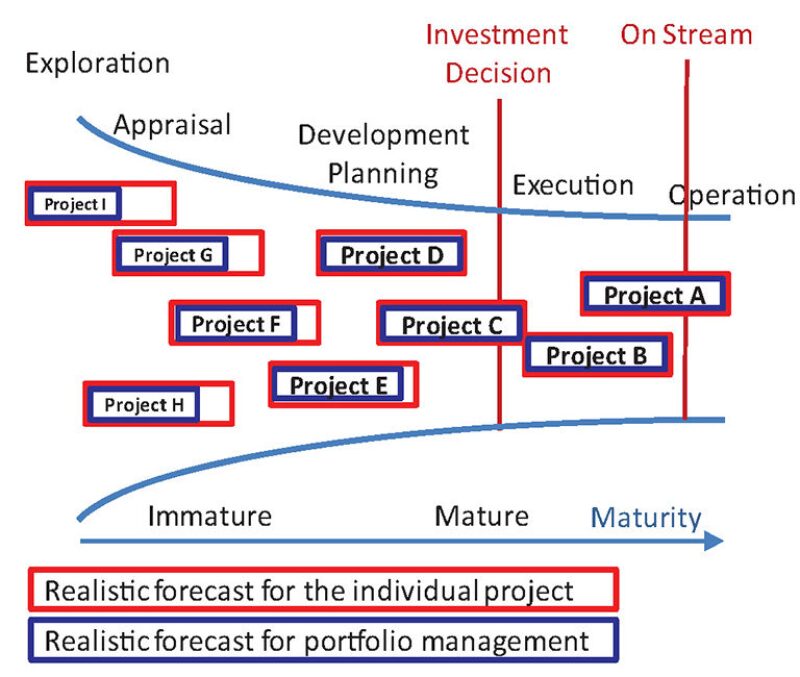
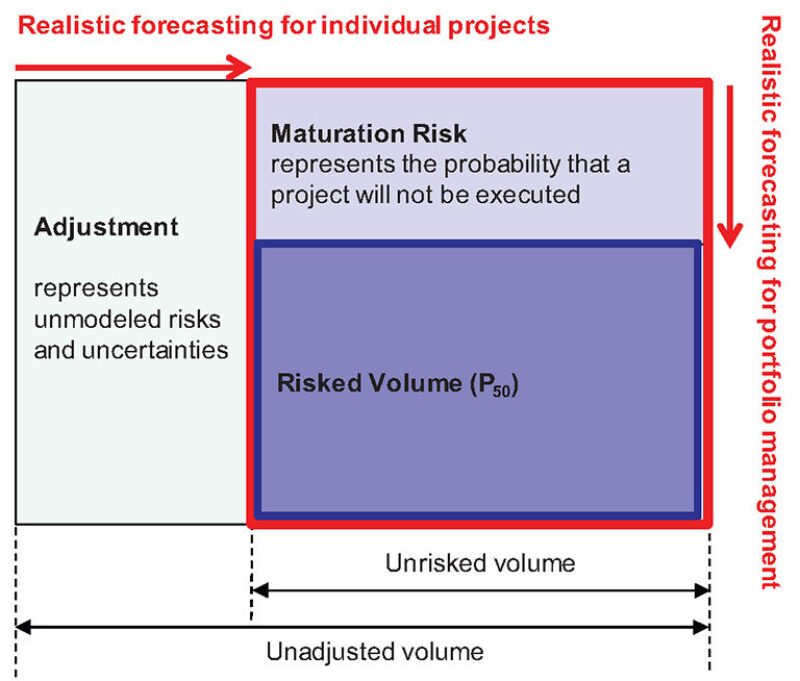
Pitfalls of Production Forecasting
Despite considerable efforts to prepare production forecasts, gaps between the forecast and the actual production are found in the majority of cases. Analysis of these gaps for a large number of E&P projects led to the following generic issues.
Unrealistic Forecast Assumptions. The assumptions used for dynamic modeling are frequently either optimistic or lack realism, resulting in over- or underprediction of the production profiles. Project schedules often assume flawless execution and ignore or underestimate the potential for delays and unexpected events. In reality, however, there is a high probability of activity slippage during drilling, hookups, construction, and startup of new facilities.
Forecasters quite often assume that operational efficiency will be 100% during the life cycle of the project. However, significant deferments are seen because of operational constraints of various facilities during the production cycle.
Human Bias. Forecasters and decision makers tend to be optimistic about their capability to judge outcomes of uncertain situations. They may underestimate uncertainty and have positively biased expectations about the consequences of their actions. They may ignore risks or underestimate the effect of risks on the forecast.
A tendency toward overconfidence may lead to overlooking certain realities. If a forecaster interprets data with a bias to confirm preconceived notions, it may result in an unrealistic outcome.
Reservoir-Modeling Limitations. Numerical simulation models approximate the production mechanisms of complex hydrocarbon accumulations. They suffer from inherent limitations in capturing the physical properties of reservoirs, wells, and process facilities. Simplified models can overpredict production because actual geology and physics are often more complex than can be captured realistically in a numerical model.
Instilling Realism in Production Forecasts
The following suggestions are useful for instilling realism in forecasts.
Realistic Forecast Assumptions. A base-case forecast should be founded on realistic assumptions about reservoir performance, project schedule, equipment availability, and production-system constraints.
The base-case-activity schedule should not assume flawless execution across all project stages. Benchmarking the proposed schedule either against an actual project track record or against a schedule of analogous projects should guide timelines for facility project completion, well delivery, and production ramp up. When selecting a base-case schedule, forecasters should examine whether the project is realistically achievable in the defined period and whether there is a 50% chance to beat the assumed schedule.
Neither perfect operational performance nor high initial uptimes for major new facilities should be assumed.
Forecast Reality Check. One should compare the forecasts with actual production on a regular basis. Reasons for significant deviations from forecasted performance should be investigated. When comparing forecasts with actual production, the essential first step is to understand any deviation in the implementation of the plan.
Forecast assumptions and uncertainty ranges should be adjusted, taking into account latest production data, drilling results, and study outcomes. In the case of project slippage, the forecast should be shifted toward the future.
Adjustment of Production Forecasts. Despite extensive technical work, production may be over- or underpredicted, particularly when forecasts are generated from dynamic models. Most of the time, it is overpredicted. Therefore, there is a requirement to adjust the simulation output to enhance the reliability of the forecasts. In the majority of cases, this adjustment will be a downward revision of the model forecast. However, there may be instances where an upward revision is needed.
Ideally, known uncertainties and risks should be captured in the dynamic model. Downward adjustment should address any residual risks and uncertainties that could not be covered in the modeling.
Model-derived forecasts can be conditioned by use of one or more of the following techniques.
Calibration Against Historical Performance. Model predictions should always be benchmarked against available field-performance data to ensure that production forecasts are realistic. Appropriate adjustment factors can be estimated from the gaps between simulation forecasts and actual performance.
Actual production achieved for recent wells is generally an excellent benchmark when forecasting initial rates and ultimate recoveries for future wells.
Analogs. It is impossible to create a 100% accurate single deterministic reservoir model. Uncertainties in the production forecast will remain even when an excellent history match is obtained.
Analogs can assist in estimating well productivity and ultimate recovery for reservoirs with limited data. When comparing recovery efficiencies predicted from a simulation study with that of an analog, it is important to identify those factors that control reservoir behavior. Specific reservoir classes can be inspected for possible analogs, such as carbonate reservoirs under waterflood.
Sensitivity Analysis. Sensitivity analysis is used to determine which model parameters have the largest effect on the history match as well as on the predicted production and recovery. Some sensitivity parameters may have little effect during the historical period but have considerable effect on long-term predictions. This knowledge is valuable in estimating a range of uncertainty for the predicted reservoir performance.
Most likely, there are multiple parameters causing uncertainty in the forecast. The following steps are recommended:
- Identify the parameters that have the largest effect on the forecast. Check whether these parameters are interdependent. If parameters are interdependent, then a simple product cannot be applied and only the parameter having the greatest effect should be chosen.
- Estimate forecast-adjustment percentage for each of these (independent) parameters. For example, Adjustment 1=–20%, Adjustment 2=–15%.
- The total adjustment will be the individual adjustments applied in succession. Using the preceding example, the total adjustment will thus be calculated from (1-20%). (1-15%)=68%, implying a total adjustment of –32%.
Summary
To enhance the reliability of a production forecasts, the following suggestions are offered:
- Be realistic in assumptions.
- Be aware of reservoir-modeling limitations.
- Carry out regular forecast reality checks.
- Adjust the simulation-derived forecast by use of some of the practical techniques explained in this paper.
- Get an external review of the forecasts.
- Do not use simulation output without considering appropriate adjustments. Never treat simulation models as accurate predictors of reservoir performance.
- Avoid bias in sharing information, during analysis, and when selecting and reporting.
- No arbitrary factor should be applied to adjust the forecasts.
This article, written by Editorial Manager Adam Wilson, contains highlights of paper SPE 155443, “Instilling Realism in Production Forecasting: Dos and Don’ts,” by Avnish K. Rajvanshi, SPE, Robert Gmelig Meyling, and Danny ten Haaf, SPE, Petroleum Development Oman, prepared for the 2012 SPE Annual Technical Conference and Exhibition, San Antonio, Texas, USA, 8–10 October. The paper has not been peer reviewed.