The authors of the complete paper write that the realm of reservoir engineering is considered to belong to the small-data regime because of the complex physical systems involved and the inherent uncertainty of the problems. The objective of the paper is to present a physics-informed neural network (PINN) technique that is able to use information from the fluid-flow physics and observed data to model the Buckley‑Leverett problem.
Fractional-Flow Theory
Through their study of fluid displacement in sands, Buckley and Leverett introduced the fractional-flow theory. The theory estimates the rate at which one fluid displaces another and, consequently, the change in fluid saturations. The theory has been applied to many fluid-flow processes in porous media such as waterflooding, carbonated waterflooding, alcohol flooding, miscible flooding, steam flooding, foam flooding, low-salinity waterflooding, and carbon sequestration.
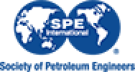