The optimization algorithm used in this work is a hybrid genetic algorithm (HGA), which is the combination of GAs with artificial neural networks (ANNs) and evolution strategies (ESs). This HGA attempts to simplify the complex and diverse parameters governing the production-optimization problem. The HGA is coupled with a commercial simulator and has been applied to real fields to quantify the benefits of this HGA over a base case with the conventional GA.
Background
GAs. GAs are part of a larger group of methods in artificial intelligence (AI) called evolutionary computation. These methods are inspired by natural evolution in biology. The GA has been well-recognized as an optimization method that has the ability to work in a solution space with nonsmooth and nonlinear topology, where traditional methods generally fail.
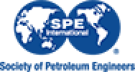