The past couple of years has been a rollercoaster for energy professionals, but it did not stop the incredible achievements in machine-learning (ML) techniques, particularly neural networks to improve seismic imaging and multiple attribute extraction to decipher reservoir rock properties and fluid contact movements in areas with data paucity and increasing difficulty in finding new or remaining hydrocarbon accumulations.
As I reviewed the seismic-related papers, I was impressed at the innovative ML methods that were developed and successfully deployed to improve already existing data and augment sometimes scarce data to aid understanding of risks and uncertainties, enhance hydrocarbon recovery, reduce cost, increase production, and, in some cases, redefine the geological understanding of the play.
Apart from the fields of seismic imaging, reservoir characterization, and hydrocarbon recovery, there were many papers devoted to new tectonic models, improved geohazards assessment, microporosity estimates, and data-acquisition methods—vertical seismic profiles, shear wave, 3D/4D ocean bottom cable, electromagnetic survey, and high-resolution broadband seismic.
It was difficult to select the top three papers for synopsis because there were so many interesting topics to choose from, but I ensured that readers receive a taste of the major topics covered, selecting some that are must-reads and recommending a few others for your reading pleasure. I hope you find them as enjoyable as I did.
This Month’s Technical Papers
Technique Improves Neural Network Training To Quantify Rock Properties From Seismic
Data-Analysis Approach Aids Reservoir-Quality Prediction of Microporous Carbonates
Seismic Work Flow Characterizes Deep, Thin Formation Layers Offshore South China Sea
Recommended Additional Reading
IPTC 19810 Hydrocarbon Exploration and Production Potential of the Gulf of Suez Basin in the Framework of the New Tectonostratigraphic Model by Janpieter Van Dijk, Dragon Oil, et al.
OTC 30620 Improving Drilling Geohazards Assessments of the Overburden Section by Performing Seismic Multiattribute Analysis by Yosmel Sanchez, Fugro, et al.
SPE 200761 Accounting for Model Errors of Rock Physics Models in 4D Seismic History-Matching Problems: A Perspective of Machine Learning by Xiaodong Luo, NORCE, et al.
SPE 202651 The Houta Prospect Hammamet Basin, Offshore Tunisia, as an Example of a New Play in an Old Basin by Janpieter van Dijk, GGS, et al.
SPE 202753 A Machine-Learning Approach to Removing Highly Variable Surface Waves for a Large Shallow-Water Survey Offshore Abu Dhabiby Al Hajeri Badreia, ADNOC, et al.
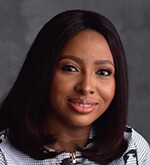
Eudorah Ochai-Audu, SPE, is onshore exploration business opportunity manager and planning lead for Shell Nigeria. She holds an MS degree in petroleum geosciences from Royal Holloway, University of London, and is a professional with the Council of Nigeria Mining Engineers and Geoscientists. Ochai-Audu joined Shell in 2008 as a trainee geoscientist. Before her current role, she was a senior production seismologist with more than 13 years of experience in 3D seismic interpretation, static reservoir modeling, and operations geology, and has been involved in several field development plans, oil and gas reservoir evaluations, and field execution projects in Nigeria and the Middle East. One of Ochai-Audu’s greatest passions is talent development, with a strong interest in career coaching and mentoring for young professionals and students. She is a strong advocate for continuous improvement, knowledge sharing, and work simplification leveraging technology. Ochai-Audu is a member of the JPT Editorial Review Committee.