Drill cuttings and core images often present classification problems. Development of an unbiased objective system that can overcome the various issues creating these difficulties is an important goal. Advances during the past decade in using convolutional neural networks (CNNs) for visual recognition of discriminately different objects means that now object recognition can be achieved to a significant extent. The benefit of such a system would be improvement of reservoir understanding by having all available images classified in a consistent manner, thus keeping characterization consistent as well.
CNNs
A CNN is a type of artificial neural network used in image recognition and processing that is specifically designed to process pixel data.
×
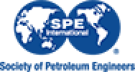
Continue Reading with SPE Membership
SPE Members: Please sign in at the top of the page for access to this member-exclusive content. If you are not a member and you find JPT content valuable, we encourage you to become a part of the SPE member community to gain full access.