The challenges of reservoir characterization can be overcome accurately and efficiently by the use of computer-based intelligence methods such as neural networks, fuzzy logic, and genetic algorithms. This paper will describe how one integrates a comprehensive methodology of data-mining techniques and artificial neural networks (ANNs) in reservoir-petrophysical-properties prediction and regeneration.
Introduction
ANNs—machine-learning models that provide the potential to establish multidimensional, nonlinear, and complex models—can be powerful tools with which to analyze experimental, industrial, and field data.
It is crucial to find the optimal data from one well to build the model with ANNs for pseudowell-log generation of a target well. Manual stratigraphic interpretation, though labor-intensive, is regarded as one approach. Data-mining techniques are another applicable approach, involving the automatic processing of data associated with nonlinearity by use of a statistical method to discover data patterns.
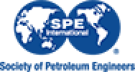