This is the fourth in a series of six articles on SPE’s Grand Challenges in Energy, formulated as the output of a 2023 workshop held by the SPE Research and Development Technical Section in Austin, Texas.
Described in a JPT article last year, each of the challenges are discussed separately in this series: geothermal energy; net-zero operations; improving recovery from tight/shale resources; digital transformation; carbon capture, utilization, and storage; and education and advocacy.
The interval from the oil price decline of 2014–2015 through the global COVID-19 pandemic of 2020–2022 was a difficult period for the upstream oil and gas business. The industry has responded, as so many times before, by seeking to reduce structural costs with the minimum impact to required new investments, including those in nondiscretionary domains (security, including cybersecurity, and safety, health, and environmental performance) as well as those required to appropriately hedge against the emergence of new, more sustainable energy sources. Digital transformation is one of the key opportunities for the industry to systematically reduce costs across multiple business domains.
While there has been active discussion of the “digital oil field” for several decades, the economic shock of the past decade revived interest in the application of digital technologies across the enterprise to improve the cost structure of businesses.
Of course, at the same time, entirely new industries, such as social media, have emerged exploiting new digital technologies, including machine learning, web software and connectivity, and cloud storage and computing. These technologies have also transformed traditional industries such as retail and manufacturing. But taking the same path in the upstream industry has some special challenges and opportunities.
This review focuses on digital transformation opportunities that are, to a greater or lesser degree, unique to the special data and operating environment of the modern petroleum industry. There are many valuable opportunities, including business process automations, that can and should be adopted by upstream companies, but are not different in kind from such innovations in other industries.
Data Is Not the New Oil
One challenge is the sheer mass and heterogeneity of upstream data. The upstream oil and gas industry is an old industry, with both business and technical records stretching back for many companies to the 19th century. These can be in paper form or a variety of digital forms reflecting the different methods of storing data since computers became a standard business and scientific tool in the 1950s. Conversion of this data to more modern formats suitable for cloud storage, for example, is not cost-free.
Thus, a key principle in digital transformation is that it must be value-driven. A frequently used phrase, “data is the new oil,” is, at least for the oil and gas business itself, deeply misleading. Most of the data held by any enterprise, be it in libraries, shared data servers, or personal hard drives, is not economically useful. Much other data, while potentially valuable, requires such substantial spending on quality assurance, data curation, data transfer to points of computation, and use of (highly paid) domain experts to interpret data analysis and make business decisions that there is no pathway to reliable value based on the data.
Instead of starting with data, the best way to think of digital transformation is to start at the other end with business decisions. Business decisions can often be improved based on superior access to and analysis of data, or the costs of certain activities can be substantially reduced. This requires the close collaboration of IT specialists, data scientists, and domain experts.
Maintenance workflows are an example. Maintenance of highly distributed facilities ideally optimizes skilled workforce activities against the likelihood of a failure occurring in the facility and the resulting operational and economic impact of that failure. Maintenance records are often poorly catalogued and may even include (even in 2024) paper records or digital records including vague or incomprehensible fields.
There can be significant value realized in using digitized records to high grade certain types of maintenance activities using actual asset performance and a strong data set of failure types and probabilities. This has been known since the dawn of the Industrial Age (if not before), but digital records, cloud storage, and access allow further optimization and careful targeting (and potentially reduction) of maintenance workforces. One common example is the use of data analytics to support predictive maintenance, in which failures or anomalies are predicted statistically, thereby allowing “just-in-time” maintenance, rather than scheduled maintenance.
A notable feature of this example is that it does not necessarily depend on highly sophisticated statistical or machine-learning methods. More important than such methods is access to properly quality-assured curated and complete data sets, covering as long a time series and as broad a geographical domain as possible. Some of this is the normal focus of an IT function, but IT investments and management need to be balanced by appropriate business ownership of the actual data and strong governance processes, defining business accountability for data quality.
In considering data architectures to support improved business results for maintenance or other domains, it is important to distinguish between two types of data systems. The more traditional data system is the “system of record,” which contains the “single source of truth” for particular instances of operational, subsurface, or financial data.
The desire to broaden the scope of analytics workflows has led to the emergence of the “system of reference,” sometimes referred to as a “system of engagement,” which is often cloud-hosted (Fig. 1). The system of reference contains data sourced from one or more systems of record and presented in an architecture that supports fast-read access, and hence enables sophisticated big data analytics. Multiple technology companies provide software and services to support the pipelining of data into systems of reference, often allowing multiple opportunities to curate and quality-assure the data.
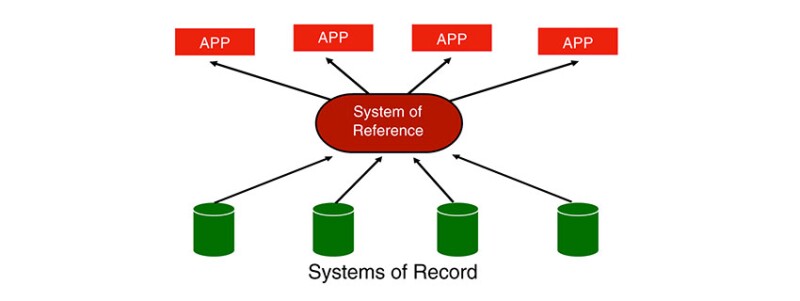
Special Features of Upstream Data
A complication in the upstream business is the fact that often data, especially subsurface data such as raw or processed seismic records, is not owned by the operating company but rather by a host government, which can impose access and data-localization requirements driven by their own sovereign interest. Thus, there may be systems of record whose contents are not appropriate to be pipelined to a system of reference that is hosted or can be easily utilized outside of a particular country. Careful consideration of legal and business requirements is needed to design the data architecture of any global upstream enterprise.
Another complication that distinguishes the upstream industry from many other industries is that data in the upstream is not necessarily cheap but is often precious and expensive. This is obviously true for purely observational data such as the results of seismic surveys but can also be true for operational data from remote or hostile environments.
When a downhole pressure sensor in a deepwater well malfunctions or “goes dark,” replacement of the sensor requires an expensive workover, which is unlikely to be economical simply to replace the sensor. A straightforward application of analytics is to reconstruct the most likely time series of data from dark sensors based on asset properties and remaining available data.
The complexity of subsurface data (seismic, well logs, reservoir models) and the confusing multitude of proprietary and open formats for such data has led to a broad initiative within the industry, the OSDU Forum, which brings together operators, service companies, and technology companies in the creation of a technology-agnostic open source data platform, with multiple cloud implementations. Originally focused on the upstream oil and gas industry, the OSDU Forum has expanded to cover downstream data as well as moving toward energy transition topics and the provision of integrated energy services.
The largest data sets routinely used in the upstream industry are seismic data sets—either raw or processed, these data sets can easily run to terabytes or petabytes. Seismic data management has been a specialized and highly technical discipline for many decades, and the advent of advanced processing techniques in the past 2 decades such as reverse time migration and full wavefield imaging has caused the upstream industry to reclaim its place as one of the primary industrial users of high-performance computing. The interpretation of this data is centered in a specialized software industry focused on seismic interpretation, reservoir modeling, and ultimately reservoir simulation. While these are not new disciplines, the advent of new technologies such as cloud computing increases opportunities to derive value from multiple data sets studied in an integrated manner.
These data sets have also provided an application for more modern machine-learning technologies such as convolutional neural nets (CNNs). A keystone application for these neural nets is pattern recognition—these nets are famous for being able to detect automatically whether photos found on the internet are photos of particular objects (e.g., cats or dogs), a capability known as classification. The success of these nets in solving classification problems has led to their application in seismic interpretation to detect particular features in a 3D seismic image, such as faults or salt bodies (Fig. 2).
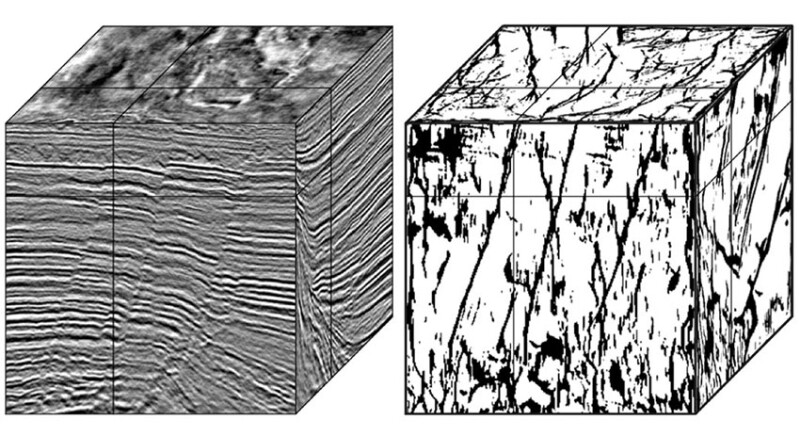
The economic impact of these innovations can be simply increased productivity for interpreters or, more ambitiously, developing systems that can detect subtle signs of hydrocarbon accumulations that might be missed by human interpreters.
Physics, Data, and the Upstream
For over a century, the upstream industry has been developing powerful physics-based methods to analyze drilling, completions, reservoir flow, and fluid behavior in tubulars and facilities. Adding these to the use of sophisticated geological and geophysical methods in exploration, the industry has a strong claim to be one of the most science-based of modern industries.
Modern statistical learning or machine-learning (ML) approaches have their roots in statistics, which has always been a key tool of engineering. The explosion in the application of these methods in the past decade or two has been more driven by the ability to collate and access large quantities of data than by underlying advances in the statistical methods themselves, which have developed more incrementally. Exceptions to this somewhat sweeping statement include CNNs as discussed above and the recent breakthrough in large language models (LLMs), of which more below.
The reader will note a philosophical difference between physics-based approaches, which exploit our scientific understanding of fundamental processes, and data-based approaches, which generate insights from the statistical manipulation of large amounts of data. An ideal would be to use a combination of these approaches which honored our hard-won knowledge of fundamental science and also exploited the abundance of data that is often available about our systems.
An interesting example of this hybridization emerges from history matching, a standard workflow in which reservoir models are modified to better match pressure and flow histories from producing wells. The use of advanced ML methods to support reservoir modeling and better understand how their structure is constrained by data has become an academic industry of its own, but unfortunately not one that has yet had a major impact on industry practice.
Another example is the use of real-time drilling data, combined with physical models of drillstring vibrational modes, to optimize drilling (Fig. 3). Here, instead of machine learning, the drillers are exploiting the rich real-time data available at a modern drilling site to achieve step changes in penetration rate.
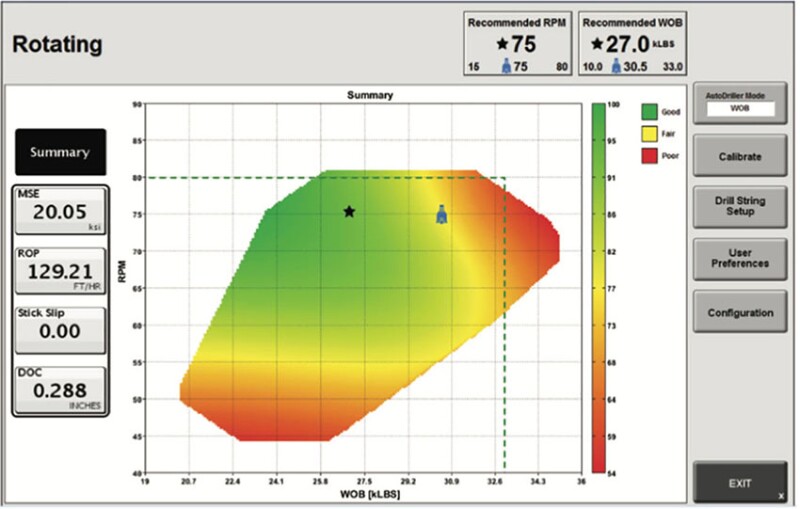
Such optimization use cases are one of the main early-value opportunities for digital transformation in the upstream. The machine-learning component of such innovations need not be terribly sophisticated—the key is to combine sound physical models with the rich data sources available in modern operations.
Beyond Optimization
Optimization is important and, when applied in a consistent and disciplined way across business operations and processes, can materially improve the competitiveness of an enterprise. But the recent excitement about breakthroughs in artificial intelligence (AI) represented by ChatGPT and its successors, large language models, raises the question of whether there may be more disruptive innovation opportunities in the upstream arising from AI. These models are based on the statistical determination of correlations between (usually) words in text documents, trained by the access to enormous amounts of textual data available through the Internet.
A natural extension of this idea would be a ChatGPT-like program trained on text and nontextual data (seismic, well logs, geological observations) to answer queries about the likelihood of exploration-relevant geological features (petroleum accumulations, source rock) being situated in particular spatial locations.
Efforts have been made to create such systems or simpler versions.Of course, an actual decision to drill an exploration well is also influenced by a variety of geopolitical, economic, regulatory, and bureaucratic factors that may not be fully captured in a corpus of geological information and may be difficult to capture at all. But skeptics of the power of LLMs have already been proven wrong in a number of cases, so we need to keep an open mind about the power of this technology.
Another interesting example is the seismic-to-simulation workflow, which moves from subsurface data through reservoir modeling and simulation with the ultimate business goal of designing and evaluating development plans for a hydrocarbon resource. This workflow, as currently practiced, is a highly complex interaction of numerous different types of software applications combined with expert input from multiple disciplines of geoscience and engineering. It is expensive, time-consuming, and a considerable source of delay between first hydrocarbon discovery and ultimate economic exploitation of a resource. This is thus a workflow ripe for disruption by new technologies emerging from AI, and there may already be companies working out of garages in Houston, Aberdeen, or Bengaluru that will succeed in doing so.
Innovation in these latter two areas has, perhaps, slowed with the general slowing of investment in exploration and development in the upstream industry. This is a paradoxical time in our industry, largely due to the push and pull of economic and political factors that create uncertainty about the pace of an energy transition driven largely by climate concerns.
Most of the comments in this review can apply equally well to new energy technologies in renewables, geothermal energy, or carbon capture and storage, especially insofar as these technologies also consume subsurface data, with all its power and eccentricity. So, whatever our future energy story may be, digital transformation will almost certainly be an important component of the narrative.
For Further Reading
Seismic Fault Detection With Convolutional Neural Network by W. Xiong, Aramco Asia; Y. Ma, Saudi Aramco; and Y. Wang, Aramco Asia, et al. SEG GEOPHYSICS.
SPE 187447 Challenges and Lessons From Implementing a Real-Time Drilling Advisory System by B.J. Spivey, G.S. Payette, and L. Wang, ExxonMobil Upstream Research Co., et al.
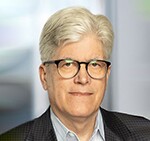
Thomas C. Halsey worked for ExxonMobil in a variety of research, management, and staff positions in Texas and New Jersey for more than 26 years, retiring as chief computational scientist in 2021. He is currently professor in the practice in the Department of Chemical and Biomolecular Engineering at Rice University.